Statistical significance for hierarchical clustering
- PMID: 28099990
- PMCID: PMC5708128
- DOI: 10.1111/biom.12647
Statistical significance for hierarchical clustering
Abstract
Cluster analysis has proved to be an invaluable tool for the exploratory and unsupervised analysis of high-dimensional datasets. Among methods for clustering, hierarchical approaches have enjoyed substantial popularity in genomics and other fields for their ability to simultaneously uncover multiple layers of clustering structure. A critical and challenging question in cluster analysis is whether the identified clusters represent important underlying structure or are artifacts of natural sampling variation. Few approaches have been proposed for addressing this problem in the context of hierarchical clustering, for which the problem is further complicated by the natural tree structure of the partition, and the multiplicity of tests required to parse the layers of nested clusters. In this article, we propose a Monte Carlo based approach for testing statistical significance in hierarchical clustering which addresses these issues. The approach is implemented as a sequential testing procedure guaranteeing control of the family-wise error rate. Theoretical justification is provided for our approach, and its power to detect true clustering structure is illustrated through several simulation studies and applications to two cancer gene expression datasets.
Keywords: High-dimension; Hypothesis testing; Multiple correction; Unsupervised learning.
© 2017, The International Biometric Society.
Figures
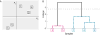
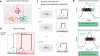
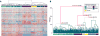
Similar articles
-
Semi-supervised adaptive-height snipping of the hierarchical clustering tree.BMC Bioinformatics. 2015 Jan 16;16(1):15. doi: 10.1186/s12859-014-0448-1. BMC Bioinformatics. 2015. PMID: 25592847 Free PMC article.
-
Spatial clustering of array CGH features in combination with hierarchical multiple testing.Stat Appl Genet Mol Biol. 2010;9:Article40. doi: 10.2202/1544-6115.1532. Epub 2010 Nov 16. Stat Appl Genet Mol Biol. 2010. PMID: 21126231
-
Fast tree aggregation for consensus hierarchical clustering.BMC Bioinformatics. 2020 Mar 20;21(1):120. doi: 10.1186/s12859-020-3453-6. BMC Bioinformatics. 2020. PMID: 32197576 Free PMC article.
-
Semisupervised Clustering by Iterative Partition and Regression with Neuroscience Applications.Comput Intell Neurosci. 2016;2016:4037380. doi: 10.1155/2016/4037380. Epub 2016 Apr 26. Comput Intell Neurosci. 2016. PMID: 27212939 Free PMC article. Review.
-
HCsnip: An R Package for Semi-supervised Snipping of the Hierarchical Clustering Tree.Cancer Inform. 2015 Mar 22;14:1-19. doi: 10.4137/CIN.S22080. eCollection 2015. Cancer Inform. 2015. PMID: 25861213 Free PMC article. Review.
Cited by
-
Proteomics and Bioinformatics Analysis of Cartilage in Post-Traumatic Osteoarthritis in a Mini-Pig Model of Anterior Cruciate Ligament Repair.Med Sci Monit. 2020 Jan 9;26:e920104. doi: 10.12659/MSM.920104. Med Sci Monit. 2020. PMID: 31916546 Free PMC article.
-
Metabolomics and Multi-Omics Integration: A Survey of Computational Methods and Resources.Metabolites. 2020 May 15;10(5):202. doi: 10.3390/metabo10050202. Metabolites. 2020. PMID: 32429287 Free PMC article. Review.
-
A systematic review of illness representation clusters in chronic conditions.Res Nurs Health. 2020 Jun;43(3):241-254. doi: 10.1002/nur.22013. Epub 2020 Feb 17. Res Nurs Health. 2020. PMID: 32067248 Free PMC article.
-
Selective Inference for Hierarchical Clustering.J Am Stat Assoc. 2024;119(545):332-342. doi: 10.1080/01621459.2022.2116331. Epub 2022 Oct 11. J Am Stat Assoc. 2024. PMID: 38660582 Free PMC article.
-
Comparison of Unsupervised Machine Learning Approaches for Cluster Analysis to Define Subgroups of Heart Failure with Preserved Ejection Fraction with Different Outcomes.Bioengineering (Basel). 2022 Apr 16;9(4):175. doi: 10.3390/bioengineering9040175. Bioengineering (Basel). 2022. PMID: 35447735 Free PMC article.
References
-
- Baik J, Silverstein JW. Eigenvalues of large sample covariance matrices of spiked population models. Journal of Multivariate Analysis. 2006;97:1382–1408.
-
- Borysov P, Hannig J, Marron JS. Asymptotics of hierarchical clustering for growing dimension. Journal of Multivariate Analysis. 2014;124:465–479.
MeSH terms
Grants and funding
LinkOut - more resources
Full Text Sources
Other Literature Sources