Unsupervised Event Characterization and Detection in Multichannel Signals: An EEG application
- PMID: 27120605
- PMCID: PMC4851103
- DOI: 10.3390/s16040590
Unsupervised Event Characterization and Detection in Multichannel Signals: An EEG application
Abstract
In this paper, we propose a new unsupervised method to automatically characterize and detect events in multichannel signals. This method is used to identify artifacts in electroencephalogram (EEG) recordings of brain activity. The proposed algorithm has been evaluated and compared with a supervised method. To this end an example of the performance of the algorithm to detect artifacts is shown. The results show that although both methods obtain similar classification, the proposed method allows detecting events without training data and can also be applied in signals whose events are unknown a priori. Furthermore, the proposed method provides an optimal window whereby an optimal detection and characterization of events is found. The detection of events can be applied in real-time.
Keywords: EEG; artifacts; event characterization; event detection; unsupervised classification.
Figures
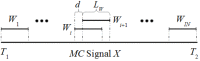
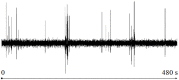
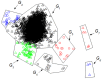
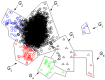
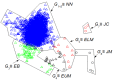
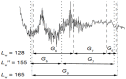
Similar articles
-
An Unsupervised Method for Artefact Removal in EEG Signals.Sensors (Basel). 2019 May 18;19(10):2302. doi: 10.3390/s19102302. Sensors (Basel). 2019. PMID: 31109062 Free PMC article.
-
An unsupervised eye blink artifact detection method for real-time electroencephalogram processing.Physiol Meas. 2016 Mar;37(3):401-17. doi: 10.1088/0967-3334/37/3/401. Epub 2016 Feb 18. Physiol Meas. 2016. PMID: 26888113
-
An Unsupervised Multichannel Artifact Detection Method for Sleep EEG Based on Riemannian Geometry.Sensors (Basel). 2019 Jan 31;19(3):602. doi: 10.3390/s19030602. Sensors (Basel). 2019. PMID: 30709001 Free PMC article.
-
Methods for artifact detection and removal from scalp EEG: A review.Neurophysiol Clin. 2016 Nov;46(4-5):287-305. doi: 10.1016/j.neucli.2016.07.002. Epub 2016 Oct 15. Neurophysiol Clin. 2016. PMID: 27751622 Review.
-
Role of EEG as biomarker in the early detection and classification of dementia.ScientificWorldJournal. 2014;2014:906038. doi: 10.1155/2014/906038. Epub 2014 Jun 30. ScientificWorldJournal. 2014. PMID: 25093211 Free PMC article. Review.
Cited by
-
Consecutive Image Acquisition without Anomalies.Sensors (Basel). 2024 Oct 14;24(20):6608. doi: 10.3390/s24206608. Sensors (Basel). 2024. PMID: 39460088 Free PMC article.
-
Automatic bad channel detection in intracranial electroencephalographic recordings using ensemble machine learning.Clin Neurophysiol. 2018 Mar;129(3):548-554. doi: 10.1016/j.clinph.2017.12.013. Epub 2017 Dec 24. Clin Neurophysiol. 2018. PMID: 29353183 Free PMC article.
-
An Unsupervised Method for Artefact Removal in EEG Signals.Sensors (Basel). 2019 May 18;19(10):2302. doi: 10.3390/s19102302. Sensors (Basel). 2019. PMID: 31109062 Free PMC article.
-
Towards Building a Computer Aided Education System for Special Students Using Wearable Sensor Technologies.Sensors (Basel). 2017 Feb 8;17(2):317. doi: 10.3390/s17020317. Sensors (Basel). 2017. PMID: 28208734 Free PMC article.
-
A Neural Algorithm for the Detection and Correction of Anomalies: Application to the Landing of an Airplane.Sensors (Basel). 2022 Mar 17;22(6):2334. doi: 10.3390/s22062334. Sensors (Basel). 2022. PMID: 35336505 Free PMC article.
References
-
- Lance B.J., Kerick S.E., Ries A.J., Oie K.S., McDowell K. Brain-computer interface technologies in the coming decades. Proc. IEEE. 2012;100:1585–1599. doi: 10.1109/JPROC.2012.2184830. - DOI
Publication types
MeSH terms
LinkOut - more resources
Full Text Sources
Other Literature Sources