A Machine Learning Approach to Bridge-Damage Detection Using Responses Measured on a Passing Vehicle
- PMID: 31546759
- PMCID: PMC6767673
- DOI: 10.3390/s19184035
A Machine Learning Approach to Bridge-Damage Detection Using Responses Measured on a Passing Vehicle
Abstract
This paper proposes a new two-stage machine learning approach for bridge damage detection using the responses measured on a passing vehicle. In the first stage, an artificial neural network (ANN) is trained using the vehicle responses measured from multiple passes (training data set) over a healthy bridge. The vehicle acceleration or Discrete Fourier Transform (DFT) spectrum of the acceleration is used. The vehicle response is predicted from its speed for multiple passes (monitoring data set) over the bridge. Root-mean-square error is used to calculate the prediction error, which indicates the differences between the predicted and measured responses for each passage. In the second stage of the proposed method, a damage indicator is defined using a Gaussian process that detects the changes in the distribution of the prediction errors. It is suggested that if the bridge condition is healthy, the distribution of the prediction errors will remain low. A recognizable change in the distribution might indicate a damage in the bridge. The performance of the proposed approach was evaluated using numerical case studies of vehicle-bridge interaction. It was demonstrated that the approach could successfully detect the damage in the presence of road roughness profile and measurement noise, even for low damage levels.
Keywords: artificial neural network; bridge; damage detection; drive-by; machine learning.
Conflict of interest statement
The authors declare no conflict of interest.
Figures
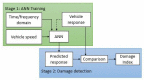
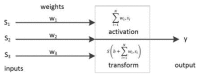
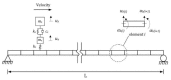
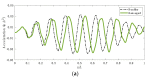
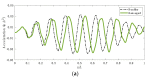
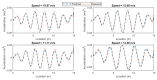
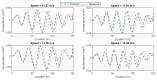
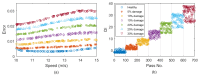
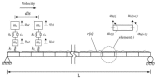
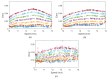
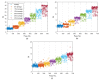
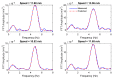
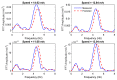
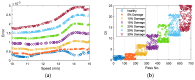
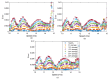
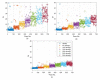
Similar articles
-
A Machine-Learning-Based Approach for Railway Track Monitoring Using Acceleration Measured on an In-Service Train.Sensors (Basel). 2023 Aug 31;23(17):7568. doi: 10.3390/s23177568. Sensors (Basel). 2023. PMID: 37688026 Free PMC article.
-
A Mobile Sensing Framework for Bridge Modal Identification through an Inverse Problem Solution Procedure and Moving-Window Time Series Models.Sensors (Basel). 2023 May 28;23(11):5154. doi: 10.3390/s23115154. Sensors (Basel). 2023. PMID: 37299882 Free PMC article.
-
A Time-Domain Signal Processing Algorithm for Data-Driven Drive-by Inspection Methods: An Experimental Study.Materials (Basel). 2023 Mar 26;16(7):2624. doi: 10.3390/ma16072624. Materials (Basel). 2023. PMID: 37048918 Free PMC article.
-
Drive-By Bridge Frequency Identification under Operational Roadway Speeds Employing Frequency Independent Underdamped Pinning Stochastic Resonance (FI-UPSR).Sensors (Basel). 2018 Nov 30;18(12):4207. doi: 10.3390/s18124207. Sensors (Basel). 2018. PMID: 30513669 Free PMC article.
-
Vehicle-Assisted Techniques for Health Monitoring of Bridges.Sensors (Basel). 2020 Jun 19;20(12):3460. doi: 10.3390/s20123460. Sensors (Basel). 2020. PMID: 32575359 Free PMC article. Review.
Cited by
-
ADAPTS: An Intelligent Sustainable Conceptual Framework for Engineering Projects.Sensors (Basel). 2020 Mar 11;20(6):1553. doi: 10.3390/s20061553. Sensors (Basel). 2020. PMID: 32168788 Free PMC article.
-
Structural Damage Diagnosis-Oriented Impulse Response Function Estimation under Seismic Excitations.Sensors (Basel). 2019 Dec 9;19(24):5413. doi: 10.3390/s19245413. Sensors (Basel). 2019. PMID: 31835297 Free PMC article.
-
Investigation of Frequency-Domain Dimension Reduction for A2M-Based Bridge Damage Detection Using Accelerations of Moving Vehicles.Materials (Basel). 2023 Feb 24;16(5):1872. doi: 10.3390/ma16051872. Materials (Basel). 2023. PMID: 36902987 Free PMC article.
-
Condition Monitoring of Railway Bridges Using Vehicle Pitch to Detect Scour.Sensors (Basel). 2024 Mar 5;24(5):1684. doi: 10.3390/s24051684. Sensors (Basel). 2024. PMID: 38475220 Free PMC article.
-
Discussion on a Vehicle-Bridge Interaction System Identification in a Field Test.Sensors (Basel). 2023 Jan 3;23(1):539. doi: 10.3390/s23010539. Sensors (Basel). 2023. PMID: 36617137 Free PMC article.
References
-
- Malekjafarian A., OBrien E.J., Golpayegani F. Indirect monitoring of critical transport infrastructure: Data analysis and signal processing. In: Alavi A., Buttlar W.G., editors. Data Anlytics Applications for Smart Cities. Auerbach/CRC Press; Boca Raton, FL, USA: 2018.
-
- Malekjafarian A., McGetrick P.J., OBrien E.J. A Review of Indirect Bridge Monitoring Using Passing Vehicles. Shock Vib. 2015;2015:1–16. doi: 10.1155/2015/286139. - DOI
-
- Yang Y.B., Lin C.W., Yau J.D. Extracting bridge frequencies from the dynamic response of a passing vehicle. J. Sound Vib. 2004;272:471–493. doi: 10.1016/S0022-460X(03)00378-X. - DOI
-
- Kim C.W., Kawatani M. Challenge for a drive-by bridge inspection; Proceedings of the 10th International Conference on Structural Safety and Reliability ICOSSAR2009; Osaka, Japan. 13–17 September 2009; pp. 758–765.
-
- Yang Y.B., Chang K.C. Extracting the bridge frequencies indirectly from a passing vehicle: Parametric study. Eng. Struct. 2009;31:2448–2459. doi: 10.1016/j.engstruct.2009.06.001. - DOI
Grants and funding
LinkOut - more resources
Full Text Sources