Lateral and Longitudinal Driving Behavior Prediction Based on Improved Deep Belief Network
- PMID: 34960592
- PMCID: PMC8706022
- DOI: 10.3390/s21248498
Lateral and Longitudinal Driving Behavior Prediction Based on Improved Deep Belief Network
Abstract
Accurately predicting driving behavior can help to avoid potential improper maneuvers of human drivers, thus guaranteeing safe driving for intelligent vehicles. In this paper, we propose a novel deep belief network (DBN), called MSR-DBN, by integrating a multi-_target sigmoid regression (MSR) layer with DBN to predict the front wheel angle and speed of the ego vehicle. Precisely, the MSR-DBN consists of two sub-networks: one is for the front wheel angle, and the other one is for speed. This MSR-DBN model allows ones to optimize lateral and longitudinal behavior predictions through a systematic testing method. In addition, we consider the historical states of the ego vehicle and surrounding vehicles and the driver's operations as inputs to predict driving behaviors in a real-world environment. Comparison of the prediction results of MSR-DBN with a general DBN model, back propagation (BP) neural network, support vector regression (SVR), and radical basis function (RBF) neural network, demonstrates that the proposed MSR-DBN outperforms the others in terms of accuracy and robustness.
Keywords: deep belief network; driving behavior prediction; intelligent vehicles.
Conflict of interest statement
The authors declare no conflict of interest.
Figures
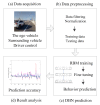
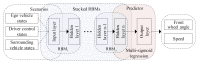
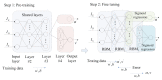
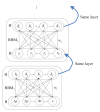
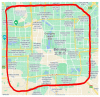
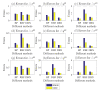
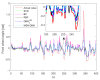
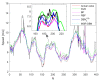
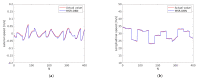
Similar articles
-
Research on imaging method of driver's attention area based on deep neural network.Sci Rep. 2022 Sep 30;12(1):16427. doi: 10.1038/s41598-022-20829-w. Sci Rep. 2022. PMID: 36180777 Free PMC article.
-
High-Resolution Neural Network for Driver Visual Attention Prediction.Sensors (Basel). 2020 Apr 4;20(7):2030. doi: 10.3390/s20072030. Sensors (Basel). 2020. PMID: 32260397 Free PMC article.
-
Vehicle Trajectory Prediction Using Hierarchical Graph Neural Network for Considering Interaction among Multimodal Maneuvers.Sensors (Basel). 2021 Aug 9;21(16):5354. doi: 10.3390/s21165354. Sensors (Basel). 2021. PMID: 34450796 Free PMC article.
-
DRER: Deep Learning-Based Driver's Real Emotion Recognizer.Sensors (Basel). 2021 Mar 19;21(6):2166. doi: 10.3390/s21062166. Sensors (Basel). 2021. PMID: 33808922 Free PMC article.
-
Modeling task-based fMRI data via deep belief network with neural architecture search.Comput Med Imaging Graph. 2020 Jul;83:101747. doi: 10.1016/j.compmedimag.2020.101747. Epub 2020 Jun 6. Comput Med Imaging Graph. 2020. PMID: 32593949 Free PMC article.
Cited by
-
On-Board Unit (OBU)-Supported Longitudinal Driving Behavior Monitoring Using Machine Learning Approaches.Sensors (Basel). 2023 Jul 27;23(15):6708. doi: 10.3390/s23156708. Sensors (Basel). 2023. PMID: 37571492 Free PMC article.
References
-
- Olabiyi O., Martinson E., Chintalapudi V., Guo R. Driver Action Prediction Using Deep (Bidirectional) Recurrent Neural Network. arXiv. 20171706.02257
-
- Sun L., Yin Y. Discovering themes and trends in transportation research using topic modeling. Transp. Res. Part C Emerg. Technol. 2017;77:49–66. doi: 10.1016/j.trc.2017.01.013. - DOI
-
- Meyer J., Becker H., Bösch P.M., Axhausen K.W. Autonomous vehicles: The next jump in accessibilities? Res. Transp. Econ. 2017;62:80–91. doi: 10.1016/j.retrec.2017.03.005. - DOI
-
- Lu C., Wang H., Lv C., Gong J., Xi J., Cao D. Learning Driver-specific Behaviour for Overtaking: A Combined Learning Framework. IEEE Trans. Veh. Technol. 2018;67:6788–6802. doi: 10.1109/TVT.2018.2820002. - DOI
-
- Schwehr J., Willert V. Driver’s gaze prediction in dynamic automotive scenes; Proceedings of the 2017 IEEE 20th International Conference on Intelligent Transportation Systems (ITSC); Yokohama, Japan. 16–19 October 2017; pp. 1–8.
MeSH terms
Grants and funding
LinkOut - more resources
Full Text Sources