Contents
Wikipedia bot wars capture the imagination of the popular press – but are they real?
- Reviewed by Aaron Halfaker (EpochFail)
A paper titled “Even Good Bots Fight: The case of Wikipedia”[1] describes a quantitative analysis of the reverting behavior of bots across different wikis. The paper has been popular in the tech media, with interviews from Dr. Yasseri (last author) appearing in Wired[supp 1], Sputnik[supp 2] and the BBC,[supp 3] among other media outlets. Regretfully, the authors failed to consider nature of “conflict” and whether it was actually conflict they were measuring, and it’s too late to get the story right in the popular press.
Through their analysis, the authors report that bots often get into “conflict”: “[…] bots on English Wikipedia reverted another bot on average 105 times, which is significantly larger than the average of 3 times for humans”. The authors assume that all revert actions represent “conflict” and conclude that the large number of reverts they discover imply “continuous disagreement” and that the activities are “inefficient as a waste of resources […]”. They observe the raw number of reverts that bots do to each other across wikis and conclude that the bots fight more in German Wikipedia than in Portuguese Wikipedia. Dr. Yasseri is quoted telling reporters for Sputnik news that “There are no normal editors looking after the work being done by these bots and this is one of the reasons of the conflict we see going on between different bots. The main reason for conflicts is lack of central supervision of bots.” This assertion, however, is dubious.
It’s too bad that Dr. Yasseri doesn’t appear to have looked into the Bot Approvals Group that oversees bot activities on English Wikipedia and the many similar groups on other wikis (e.g. the Wikipédia:Robôs/Grupo de aprovação in Portuguese Wikipedia). It would be interesting if these centralized governance strategies were ineffective at preventing bots from getting into conflict.
While reverts between human editors often do represent conflict over which content should appear in an article, the authors do not check that this assumption holds with bots. The paper contains no content analysis that might describe what these “contentious disagreements” look like, beyond a brief statement that much of the reverts happen between bots that were fixing inter-wiki links and are likely no longer a problem since the introduction of Wikidata in 2013. A cursory review of their open-licensed data release suggests that many of these bot-reverts take place years after the original bot edit — and in response to human actions like the renaming of an article (for example, when human user Nightstallion moved Mohammad Beheshti to Ayatollah Mohammad Beheshti and RussBot came to fix a redirect from Dr. Mohammad Beheshti in 2006 and then 2 years later, Mohsenkazempur moved it back and Addbot came back to fix the redirect again, that looks like a bot revert). If the authors had explored what was happening in these reverts and the mechanisms by which wiki communities observe and govern bot behaviors, they might have drawn different conclusions and not referred to this activity as a “fight” or “conflict”. While it’s certainly true that bot fights do sometimes happen, the authors don’t seem to have discovered or described any real phenomena of bot vs. bot “conflict”. If they had, they might have told a different story of how rare such fights are and how quickly they are resolved by human editors. Regretfully, it’s too late to get the story right with the popular press. “Robot wars in Wikipedia” has proven too juicy of a story to pass up.
(See also our review of a previous paper coauthored by Dr. Yasseri that likewise focused heavily on conflicts and received a large amount of media attention: “The most controversial topics in Wikipedia: a multilingual and geographical analysis”)
“Relationship between personality and attitudes to Wikipedia”
- Reviewed by Piotr Konieczny
This conference paper[2] touches upon a very interesting yet understudied question: psychological dimensions of why people contribute to Wikipedia. The topic of motivations of Wikipedia contributors has been tackled before, but not much research has focused on said psychological aspects, which promise to teach us more about differences between individuals who have potential to become volunteer contributors. The study, based on a sample of Polish students (206 University of Gdańsk students in their early 20s, over half from the pedagogics field, over 80% female), looked at six personality traits (extraversion, openness to experience, conscientiousness, agreeableness, emotional stability and cynical hostility – the first four are also a part of the Big Five personality traits). One of the authors’ goals was to test whether cynical hostility would be negatively correlated to editing Wikipedia, and to one’s opinion of it. Besides attitudes towards Wikipedia, the study also measured the students’ attitude towards traditional encyclopedias, radio, press and TV.
The authors found that conscientiousness was negatively, but weakly, related to editing Wikipedia and to positive opinions about Wikipedia. Cynical hostility was not related to any specific attitude towards Wikipedia. Extraversion and openness to experience were positively, but weakly, related to positive opinions about Wikipedia. The authors suggest that the lack of relation between cynical hostility (distrust of other people) and Wikipedia may exist partially because many students do not associate Wikipedia with the work of other individuals. They noted their findings are not consistent with prior studies; citing a study which suggested that knowledge sharing is related to openness to experience, conscientiousness and agreeableness – through noting that that study was based on sharing knowledge inside a company, an environment that is somewhat different from doing so in the public, volunteer setting of Wikipedia. At the same time, this reviewer notes that the study does not demonstrate any statistically significant Wikipedia-related correlations. Overall, it seems like an interesting study, but with statistically insignificant, inconclusive findings. Whether the studied population was too small, or too biased, is hard to say, but this reviewer hopes future studies will pursue this paper’s central question. The psychological dimension of why people contribute, like, or dislike and not contribute to Wikipedia is a very interesting issue. Even with no conclusive findings, this study shows the potential of this topic.
“ExpertIdeas: Incentivizing Domain Experts to Contribute to Wikipedia”
- Reviewed by Piotr Konieczny
It is generally known that while many experts (professors, etc.) use Wikipedia, they rarely contribute to it (which, generally, is not that different from how non-experts use but don’t contribute to it). This preprint[3] presents the results of a randomized field experiment, inspired by w:social loafing theory, investigating how different incentives could motivate experts to contribute. In the authors’ own words: “We investigate incentives that Wikipedia can provide for scholars to motivate them to contribute”. The authors (including w:User:I.yeckehzaare) are familiar enough with the Wikipedia community to be able to create and operate a bot (w:User:ExpertIdeasBot, approved by the community in 2014); additional resources about this study are available at Wikipedia:WikiProject Economics/ExpertIdeas. The authors sent a number of invitations to 3,974 researchers (from the field of economics). The bot has been operating roughly from August 2014 to December 2016. An example edit can be seen here. The paper discusses the design of the experiment, and the result, in detail, and also contains a supporting statistical analysis showing a number of significant results. The researchers expect the paper to be published in finalized form next year, and are still doing work on assessing the quality of the expert comments.
The authors conclude that experts are more likely to contribute if they receive a personalized email clearly mentioning their recent studies and areas of expertise. Another helpful aspect is if this invitation comes from an expert in the same field (rather than a random other person, including a random Wikipedia volunteer or WMF staff member). It is also helpful to appeal not only to the self-less argument that “We should contribute to Wikipedia because it is a public good, etc.”, but also to more selfish motives, such as that one can add citations to one’s own work to Wikipedia which can improve the likelihood of their publications being cited. Experts would also like for contributions to be more easily identifiable and attributable, and it is suggested that Wikipedia should make it easier for experts to receive recognition, for example through listing their contributions and names on a related WikiProject page.
Overall, this is a very interesting study, and it is commendable the authors did it in a way that is highly transparent to the community. The code for the bot is available on GitHub, though I was unable to find any indication that it is freely licensed, which sadly suggests that if the Wikipedia community would like to reuse it, it may not be able to do so (we will correct this statement as soon as any clarification/license link is found and available). Hopefully, the Wikipedia community and WMF will be able to capitalize on the findings from this study, developing it into a larger outreach program to academics.
Other recent publications
Other recent publications that could not be covered in time for this issue include the items listed below. contributions are always welcome for reviewing or summarizing newly published research.
- Compiled by Tilman Bayer
- “Crowdsourcing not all sourced by the crowd: An observation on the behavior of Wikipedia participants”[4] From the abstract: “From the analysis of 342 Wikipedia articles, this study shows that the overall tone of Wikipedia articles is mostly decided by a dominant few rather than by a trivial many, and such domination worsens as the number of participant increases and the article matures. This result contradicts a common belief on crowdsourcing that Wikipedia would reflect the voices of a vast majority, obtain a balanced solution, and attain democracy on the Internet. Therefore, this study contributes to the literature by analyzing how effectively Wikipedia functions as a crowdsourcing platform within the context. It also implies that developing a proper crowdsourcing strategy such as effective management of a platform is necessary, especially when an organization has a specific goal to achieve throughout a project.”
- “A Method for Predicting Wikipedia Editors’ Editing Interest: Based on a Factor Graph Model”[5] From the abstract: “this paper proposes an Interest Prediction Factor Graph (IPFG) model, which is characterized by editor’s social properties, hyperlinks between Wikipedia entries, the categories of an entry and other important features, to predict an editor’s editing interest in types of Wikipedia entries.”
- “Social patterns and dynamics of creativity in Wikipedia”[6] From the abstract: “We collect contribution data from a random sample of Wikipedia articles and use a novel approach of analysing the correlations between editors’ contribution patterns over the life-time of the articles. We find support for the existence of four socially conditioned personas among the editors and statistical difference in distribution of personas in articles of different qualities. Our findings add domain-specific details, features and attributes to the existing knowledge on editor roles and personas.”
- “Trusting Wikipedia. Vandalism attacks and content resilience: an analysis model and some empirical evidence” (in Italian, original title: “Fidarsi di Wikipedia. Attacchi vandalici e resilienza dei contenuti: un modello di analisi ed alcune evidenze empiriche”)[7] From the abstract (translated): “As for the resilience capacity of Wikipedia, the results are obtained using an empirical approach. This consists of inserting errors within the page sample [on the Italian WIkipedia] under specific methodological constraints and then assessing how soon and in what manner these errors are corrected.”
- “Does Wikipedia matter? The effect of Wikipedia on tourist choices”[8] From the abstract: ” Our results suggest a strong observational correlation between the amount of content on Wikipedia and tourist overnight stays. We propose a check of whether this correlation is causal. For that, we introduce randomized exogenous variation to articles’ content. While our treatment is strong enough to affect content on the treated pages positively, we find no statistically significant effect of this treatment on tourist overnight stays.”
- “Travel Attractions Recommendation with Knowledge Graphs”[9] From the abstract: “we constructed a rich world scale travel knowledge graph from existing large knowledge graphs namely Geonames, DBpedia and Wikidata. The underlying ontology contains more than 1200 classes to describe attractions. We applied a city-dependent user profiling strategy that makes use of the fine semantics encoded in the constructed graph.”
- Identifying missing topics in a knowledge graph based on Wikipedia’s notability criteria[10] From the abstract: “While large Knowledge Graphs (KGs) already cover a broad range of domains to an extent sufficient for general use, they typically lack emerging entities that are just starting to attract the public interest. This disqualifies such KGs for tasks like entity-based media monitoring, since a large portion of news inherently covers entities that have not been noted by the public before. [… We] propose a machine learning approach which tackles the most frequent but least investigated challenge, i.e., when entities are missing in the KG and cannot be considered by entity linking systems. We construct a publicly available benchmark data set based on English news articles and editing behavior on Wikipedia. Our experiments show that predicting whether an entity will be added to Wikipedia is challenging. However, we can reliably identify emerging entities that could be added to the KG according to Wikipedia’s own notability criteria.”
- “A Corpus of Wikipedia Discussions: Over the Years, with Topic, Power and Gender Label”[11] From the abstract: “we present a large corpus of Wikipedia Talk page discussions that are collected from a broad range of topics, containing discussions that happened over a period of 15 years. The dataset contains 166,322 discussion threads, across 1236 articles/topics that span 15 different topic categories or domains. The dataset also captures whether the post is made by an registered user or not, and whether he/she was an administrator at the time of making the post. It also captures the Wikipedia age of editors in terms of number of months spent as an editor, as well as their gender.”
- “Modeling user interest in social media using news media and Wikipedia”[12] From the abstract: “… we propose a user modeling framework that maps the content of texts in social media to relevant categories in news media. In our framework, the semantic gaps between social media and news media are reduced by using Wikipedia as an external knowledge base. We map term-based features from a short text and a news category into Wikipedia-based features such as Wikipedia categories and article entities. A user’s microposts are thus represented in a rich feature space of words. Experimental results show that our proposed method using Wikipedia-based features outperforms other existing methods of identifying users’ interests from social media.”
- Visualization tool for experiments with Wikipedia as a NLP resource: [13] From the abstract: “we describe Docforia, a multilayer document model and application programming interface (API) to store formatting, lexical, syntactic, and semantic annotations on Wikipedia and other kinds of text and visualize them. While Wikipedia has become a major NLP resource, its scale and heterogeneity makes it relatively difficult to do experimentations on the whole corpus. These experimentations are rendered even more complex as, to the best of our knowledge, there is no available tool to visualize easily the results of a processing pipeline…”
- “Leveraging the Crowdsourcing of Lexical Resources for Bootstrapping a Linguistic Data Cloud”[14] From the abstract: “We present a declarative approach implemented in a comprehensive open-source framework based on DBpedia to extract lexical-semantic resources – an ontology about language use – from Wiktionary. The data currently includes language, part of speech, senses, definitions, synonyms, translations and taxonomies (hyponyms, hyperonyms, synonyms, antonyms) for each lexical word. Main focus is on flexibility to the loose schema and configurability towards differing language-editions of Wiktionary. [..] The extracted data is as fine granular as the source data in Wiktionary […]. It enables use cases like disambiguation or machine translation. By offering a linked data service, we hope to extend DBpedia’s central role in the LOD infrastructure to the world of Open Linguistics.”
- “Jewish, Christian and Islamic in the English Wikipedia”[15] From the abstract: “I use corpus linguistics tools to extract the adjective noun collocates of the adjectives Jewish, Christian, and Islamic from the 2013 English Wikipedia in order find out their semantic prosody.[…] In the case of negative nouns, an ANOVA test found a statistically significant difference. Pair-wise comparisons suggest that Islamic is more negative than either Christian or Jewish … “
References
- ↑ Tsvetkova, Milena; García-Gavilanes, Ruth; Floridi, Luciano; Yasseri, Taha (2017-02-23). “Even Good Bots Fight: The case of Wikipedia”. PLOS ONE 12 (2): e0171774. doi:10.1371/journal.pone.0171774.
- ↑ Atroszko, Bartosz; Bereznowski, Piotr; Wróbel, Wiktor Kornel; Atroszko, Paweł (2016-08-08). “Relationship between personality and attitudes to Wikipedia”. The 5th Electronic International Interdisciplinary Conference. p. 6. ISBN 978-80-554-1248-1.
- ↑ Yan Chen, Rosta Farzan, Robert Kraut, Iman YeckehZaare and Ark Fangzhou Zhang: ExpertIdeas: Incentivizing Domain Experts to Contribute to Wikipedia. January 30, 2017
- ↑ Lee, Jung; Seo, DongBack. “Crowdsourcing not all sourced by the crowd: An observation on the behavior of Wikipedia participants”. Technovation. doi:10.1016/j.technovation.2016.05.002. ISSN 0166-4972.
- ↑ Zhang, Haisu; Zhang, Sheng; Wu, Zhaolin; Huang, Liwei; Ma, Yutao (2016-07-01). “A Method for Predicting Wikipedia Editors’ Editing Interest: Based on a Factor Graph Model”. International Journal of Web Services Research (IJWSR) 13 (3): 1–25. doi:10.4018/IJWSR.2016070101. ISSN 1545-7362.
- ↑ Launonen, Pentti; Tiilikainen, Sanna; Kern, K.c. (2016-01-01). “Social patterns and dynamics of creativity in Wikipedia”. International Journal of Organisational Design and Engineering 4 (1-2): 137–152. doi:10.1504/IJODE.2016.080170. ISSN 1758-9797.
- ↑ Dezaiacomo, Simone (2014-07-21). “Fidarsi di Wikipedia. Attacchi vandalici e resilienza dei contenuti: un modello di analisi ed alcune evidenze empiriche”.
- ↑ Hinnosaar, Marit; Hinnosaar, Toomas; Kummer, Michael; Slivko, Olga (2015). Does Wikipedia matter? The effect of Wikipedia on tourist choices. ZEW Discussion Papers.
- ↑ Lu, Chun; Laublet, Philippe; Stankovic, Milan (2016-11-19). “Travel Attractions Recommendation with Knowledge Graphs”. In Eva Blomqvist, Paolo Ciancarini, Francesco Poggi, Fabio Vitali (eds.). Knowledge Engineering and Knowledge Management. Lecture Notes in Computer Science. Springer International Publishing. pp. 416–431. ISBN 9783319490038.
- ↑ Färber, Michael; Rettinger, Achim; Asmar, Boulos El (2016-11-19). “On Emerging Entity Detection”. In Eva Blomqvist, Paolo Ciancarini, Francesco Poggi, Fabio Vitali (eds.). Knowledge Engineering and Knowledge Management. Lecture Notes in Computer Science. Springer International Publishing. pp. 223–238. ISBN 9783319490038.
Supplementary materials: http://people.aifb.kit.edu/he9318/emerging-entity-detection/
- ↑ Prabhakaran, Vinodkumar; Rambow, Owen (2016). “A Corpus of Wikipedia Discussions: Over the Years, with Topic, Power and Gender Labels”. p. 5.
- ↑ Kang, Jaeyong; Lee, Hyunju (April 2017). “Modeling user interest in social media using news media and wikipedia”. Information Systems 65: 52–64. doi:10.1016/j.is.2016.11.003. ISSN 0306-4379.
- ↑ Klang, Marcus; Nugues, Pierre (2016). Docforia: A Multilayer Document Model (PDF). Department of computer science Lund University, Lund. p. 4.
- ↑ Hellmann, Sebastian; Brekle, Jonas; Auer, Sören (2012-12-02). “Leveraging the Crowdsourcing of Lexical Resources for Bootstrapping a Linguistic Data Cloud”. In Hideaki Takeda, Yuzhong Qu, Riichiro Mizoguchi, Yoshinobu Kitamura (eds.). Semantic Technology. Lecture Notes in Computer Science. Springer Berlin Heidelberg. pp. 191–206. ISBN 978-3-642-37995-6.
- ↑ Mohamed, Emad (2016-12-29). “Jewish, Christian and Islamic in the English Wikipedia”. Online – Heidelberg Journal of Religions on the Internet 11 (0). doi:10.17885/heiup.rel.2016.0.23630.
- Supplementary references:
- Editorial note: Recent issues have been held up due to the situation at The Signpost, the English Wikipedia’s community newsletter, which of late has ceased publication. With apologies for the delays, we are now resuming the Wikimedia Research Newsletter while our longstanding collaboration and syndication arrangement with The Signpost is on hold. (Contributions continue to be welcome; note that we will likely produce the next issues separately on Meta-wiki instead of as a Signpost draft page.)
Wikimedia Research Newsletter
Vol: 7 • Issue: 2 • February 2017
This newsletter is brought to you by the Wikimedia Research Committee and The Signpost
Subscribe: Email
• [archives] [contribute] [research index]
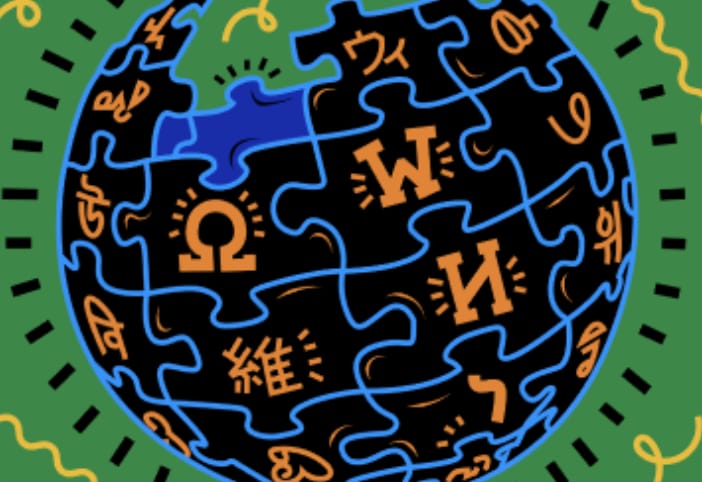
Can you help us translate this article?
In order for this article to reach as many people as possible we would like your help. Can you translate this article to get the message out?
Start translation