Source connectivity analysis with MEG and EEG
- PMID: 19235884
- PMCID: PMC6870611
- DOI: 10.1002/hbm.20745
Source connectivity analysis with MEG and EEG
Abstract
Interactions between functionally specialized brain regions are crucial for normal brain function. Magnetoencephalography (MEG) and electroencephalography (EEG) are techniques suited to capture these interactions, because they provide whole head measurements of brain activity in the millisecond range. More than one sensor picks up the activity of an underlying source. This field spread severely limits the utility of connectivity measures computed directly between sensor recordings. Consequentially, neuronal interactions should be studied on the level of the reconstructed sources. This article reviews several methods that have been applied to investigate interactions between brain regions in source space. We will mainly focus on the different measures used to quantify connectivity, and on the different strategies adopted to identify regions of interest. Despite various successful accounts of MEG and EEG source connectivity, caution with respect to the interpretation of the results is still warranted. This is due to the fact that effects of field spread can never be completely abolished in source space. However, in this very exciting and developing field of research this cautionary note should not discourage researchers from further investigation into the connectivity between neuronal sources.
(c) 2009 Wiley-Liss, Inc.
Figures
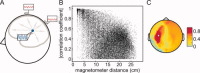
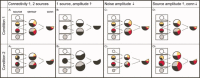
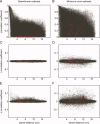
Similar articles
-
Hyperedge bundling: A practical solution to spurious interactions in MEG/EEG source connectivity analyses.Neuroimage. 2018 Jun;173:610-622. doi: 10.1016/j.neuroimage.2018.01.056. Epub 2018 Jan 31. Neuroimage. 2018. PMID: 29378318
-
Ghost interactions in MEG/EEG source space: A note of caution on inter-areal coupling measures.Neuroimage. 2018 Jun;173:632-643. doi: 10.1016/j.neuroimage.2018.02.032. Epub 2018 Feb 22. Neuroimage. 2018. PMID: 29477441
-
Phase shift invariant imaging of coherent sources (PSIICOS) from MEG data.Neuroimage. 2018 Dec;183:950-971. doi: 10.1016/j.neuroimage.2018.08.031. Epub 2018 Aug 22. Neuroimage. 2018. PMID: 30142449
-
Opportunities and methodological challenges in EEG and MEG resting state functional brain network research.Clin Neurophysiol. 2015 Aug;126(8):1468-81. doi: 10.1016/j.clinph.2014.11.018. Epub 2014 Nov 28. Clin Neurophysiol. 2015. PMID: 25511636 Review.
-
Magnetoencephalography for localizing and characterizing the epileptic focus.Handb Clin Neurol. 2019;160:203-214. doi: 10.1016/B978-0-444-64032-1.00013-8. Handb Clin Neurol. 2019. PMID: 31277848 Review.
Cited by
-
Stairway to memory: Left-hemispheric alpha dynamics index the progressive loading of items into a short-term store.Neuroimage. 2021 Jul 15;235:118024. doi: 10.1016/j.neuroimage.2021.118024. Epub 2021 Apr 6. Neuroimage. 2021. PMID: 33836267 Free PMC article.
-
Increased Intrinsic Connectivity of the Default Mode Network in Temporal Lobe Epilepsy: Evidence from Resting-State MEG Recordings.PLoS One. 2015 Jun 2;10(6):e0128787. doi: 10.1371/journal.pone.0128787. eCollection 2015. PLoS One. 2015. PMID: 26035750 Free PMC article.
-
Task-sensitive reconfiguration of corticocortical 6-20 Hz oscillatory coherence in naturalistic human performance.Hum Brain Mapp. 2015 Jul;36(7):2455-69. doi: 10.1002/hbm.22784. Epub 2015 Mar 11. Hum Brain Mapp. 2015. PMID: 25760689 Free PMC article.
-
Dynamic changes in brain functional connectivity during concurrent dual-task performance.PLoS One. 2011;6(11):e28301. doi: 10.1371/journal.pone.0028301. Epub 2011 Nov 29. PLoS One. 2011. PMID: 22140572 Free PMC article.
-
Cross-frequency power coupling between hierarchically organized face-selective areas.Cereb Cortex. 2014 Sep;24(9):2409-20. doi: 10.1093/cercor/bht097. Epub 2013 Apr 15. Cereb Cortex. 2014. PMID: 23588186 Free PMC article.
References
-
- Astolfi L,Cincotti F,Mattia D,Salinari S,Babiloni C,Basilisco A,Rossini PM,Ding L,Ni Y,He B,Marciani MG,Babiloni F ( 2004): Estimation of the effective and functional human cortical connectivity with structural equation modeling and directed transfer function applied to high‐resolution EEG. Magn Reson Imaging 22: 1457–1470. - PubMed
-
- Astolfi L,Cincotti F,Mattia D,Babiloni C,Carducci F,Basilisco A,Rossini PM,Salinari S,Ding L,Ni Y,He B,Babiloni F ( 2005): Assessing cortical functional connectivity by linear inverse estimation and directed transfer function: simulations and application to real data. Clin Neurophysiol 116: 920–932. - PubMed
-
- Astolfi L,Cincotti F,Mattia D,De Vico Fallani F,Tocci A,Colosimo A,Salinari S,Marciani MG,Hesse W,Witte H,Ursino M,Zavaglia M,Babiloni F ( 2008): Tracking the time‐varying cortical connectivity patterns by adaptive multivariate estimators. IEEE Trans Biomed Eng 55: 902–913. - PubMed
-
- Babiloni F,Cincotti F,Babiloni C,Carducci F,Mattia D,Astolfi L,Basilisco A,Rossini PM,Ding L,Ni Y,Cheng J,Christine K,Sweeney J,He B ( 2005): Estimation of the cortical functional connectivity with the multimodal integration of high‐resolution EEG and fMRI data by directed transfer function. Neuroimage 24: 118–131. - PubMed
-
- Baccala LA,Sameshima K ( 2001): Partial directed coherence: A new concept in neural structure determination. Biol Cybern 84: 463–474. - PubMed
Publication types
MeSH terms
LinkOut - more resources
Full Text Sources
Other Literature Sources