Identification of Risk Factors Associated with Obesity and Overweight-A Machine Learning Overview
- PMID: 32403349
- PMCID: PMC7248873
- DOI: 10.3390/s20092734
Identification of Risk Factors Associated with Obesity and Overweight-A Machine Learning Overview
Abstract
Social determining factors such as the adverse influence of globalization, supermarket growth, fast unplanned urbanization, sedentary lifestyle, economy, and social position slowly develop behavioral risk factors in humans. Behavioral risk factors such as unhealthy habits, improper diet, and physical inactivity lead to physiological risks, and "obesity/overweight" is one of the consequences. "Obesity and overweight" are one of the major lifestyle diseases that leads to other health conditions, such as cardiovascular diseases (CVDs), chronic obstructive pulmonary disease (COPD), cancer, diabetes type II, hypertension, and depression. It is not restricted within the age and socio-economic background of human beings. The "World Health Organization" (WHO) has anticipated that 30% of global death will be caused by lifestyle diseases by 2030 and it can be prevented with the appropriate identification of associated risk factors and behavioral intervention plans. Health behavior change should be given priority to avoid life-threatening damages. The primary purpose of this study is not to present a risk prediction model but to provide a review of various machine learning (ML) methods and their execution using available sample health data in a public repository related to lifestyle diseases, such as obesity, CVDs, and diabetes type II. In this study, we _targeted people, both male and female, in the age group of >20 and <60, excluding pregnancy and genetic factors. This paper qualifies as a tutorial article on how to use different ML methods to identify potential risk factors of obesity/overweight. Although institutions such as "Center for Disease Control and Prevention (CDC)" and "National Institute for Clinical Excellence (NICE)" guidelines work to understand the cause and consequences of overweight/obesity, we aimed to utilize the potential of data science to assess the correlated risk factors of obesity/overweight after analyzing the existing datasets available in "Kaggle" and "University of California, Irvine (UCI) database", and to check how the potential risk factors are changing with the change in body-energy imbalance with data-visualization techniques and regression analysis. Analyzing existing obesity/overweight related data using machine learning algorithms did not produce any brand-new risk factors, but it helped us to understand: (a) how are identified risk factors related to weight change and how do we visualize it? (b) what will be the nature of the data (potential monitorable risk factors) to be collected over time to develop our intended eCoach system for the promotion of a healthy lifestyle _targeting "obesity and overweight" as a study case in the future? (c) why have we used the existing "Kaggle" and "UCI" datasets for our preliminary study? (d) which classification and regression models are performing better with a corresponding limited volume of the dataset following performance metrics?
Keywords: BMI; Prisma; Sklearn; calibration; classification; data visualization; deep learning; discrimination; eCoach; gradient descent; hypothesis test; lifestyle diseases; machine learning; model performance; monitoring; normal distribution; obesity; overweight; python; regression; sensor data.
Conflict of interest statement
The authors declare no conflict of interest.
Figures
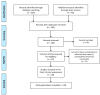
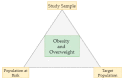
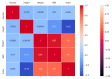
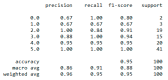
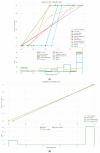
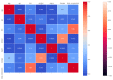
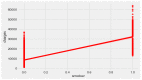
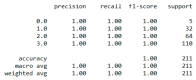
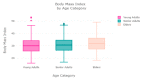
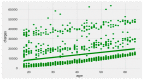
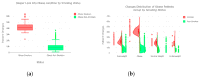
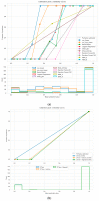
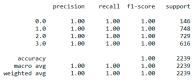
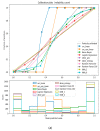
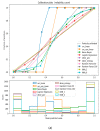
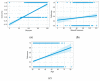
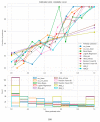
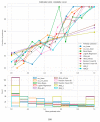
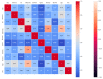
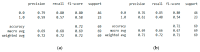
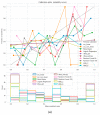
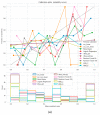
Similar articles
-
The effect of weight management interventions that include a diet component on weight-related outcomes in pregnant and postpartum women: a systematic review protocol.JBI Database System Rev Implement Rep. 2015 Jan;13(1):88-98. doi: 10.11124/jbisrir-2015-1812. JBI Database System Rev Implement Rep. 2015. PMID: 26447010
-
Using interpretable machine learning methods to identify the relative importance of lifestyle factors for overweight and obesity in adults: pooled evidence from CHNS and NHANES.BMC Public Health. 2024 Nov 1;24(1):3034. doi: 10.1186/s12889-024-20510-z. BMC Public Health. 2024. PMID: 39487401 Free PMC article.
-
A systematic literature review on obesity: Understanding the causes & consequences of obesity and reviewing various machine learning approaches used to predict obesity.Comput Biol Med. 2021 Sep;136:104754. doi: 10.1016/j.compbiomed.2021.104754. Epub 2021 Aug 16. Comput Biol Med. 2021. PMID: 34426171 Review.
-
Screening and Interventions for Childhood Overweight [Internet].Rockville (MD): Agency for Healthcare Research and Quality (US); 2005 Jul. Rockville (MD): Agency for Healthcare Research and Quality (US); 2005 Jul. PMID: 20722132 Free Books & Documents. Review.
-
[Impact of lifestyle and obesity to the risk of type 2 diabetes: a prospective study in Jiangsu province].Zhonghua Yu Fang Yi Xue Za Zhi. 2012 Apr;46(4):311-5. Zhonghua Yu Fang Yi Xue Za Zhi. 2012. PMID: 22800627 Chinese.
Cited by
-
Type D Personality and Health Behaviors in People Living with Obesity.Int J Environ Res Public Health. 2022 Nov 8;19(22):14650. doi: 10.3390/ijerph192214650. Int J Environ Res Public Health. 2022. PMID: 36429364 Free PMC article.
-
Ultra-Processed Food Intake and Increased Risk of Obesity: A Narrative Review.Foods. 2024 Aug 21;13(16):2627. doi: 10.3390/foods13162627. Foods. 2024. PMID: 39200554 Free PMC article. Review.
-
Machine learning insights concerning inflammatory and liver-related risk comorbidities in non-communicable and viral diseases.World J Gastroenterol. 2022 Nov 28;28(44):6230-6248. doi: 10.3748/wjg.v28.i44.6230. World J Gastroenterol. 2022. PMID: 36504554 Free PMC article. Review.
-
Lifestyle behaviors and stress are risk factors for overweight and obesity in healthcare workers: a cross-sectional survey.BMC Public Health. 2023 Sep 14;23(1):1791. doi: 10.1186/s12889-023-16673-w. BMC Public Health. 2023. PMID: 37710220 Free PMC article.
-
Effect of a 1-Year Controlled Lifestyle Intervention on Body Weight and Other Risk Markers (the Healthy Lifestyle Community Programme, Cohort 2).Obes Facts. 2022;15(2):228-239. doi: 10.1159/000521164. Epub 2021 Dec 17. Obes Facts. 2022. PMID: 34923493 Free PMC article. Clinical Trial.
References
-
- WHO Page. [(accessed on 18 March 2020)]; Available online: https://www.who.int/news-room/fact-sheets/detail/obesity-and-overweight; https://www.who.int/nmh/publications/ncd_report_chapter1.pdf.
-
- CDC Page. [(accessed on 18 March 2020)]; Available online: https://www.cdc.gov/obesity/adult/index.html.
MeSH terms
LinkOut - more resources
Full Text Sources
Medical