Optimizing COVID-19 surveillance in long-term care facilities: a modelling study
- PMID: 33287821
- PMCID: PMC7721547
- DOI: 10.1186/s12916-020-01866-6
Optimizing COVID-19 surveillance in long-term care facilities: a modelling study
Abstract
Background: Long-term care facilities (LTCFs) are vulnerable to outbreaks of coronavirus disease 2019 (COVID-19). Timely epidemiological surveillance is essential for outbreak response, but is complicated by a high proportion of silent (non-symptomatic) infections and limited testing resources.
Methods: We used a stochastic, individual-based model to simulate transmission of severe acute respiratory syndrome coronavirus 2 (SARS-CoV-2) along detailed inter-individual contact networks describing patient-staff interactions in a real LTCF setting. We simulated distribution of nasopharyngeal swabs and reverse transcriptase polymerase chain reaction (RT-PCR) tests using clinical and demographic indications and evaluated the efficacy and resource-efficiency of a range of surveillance strategies, including group testing (sample pooling) and testing cascades, which couple (i) testing for multiple indications (symptoms, admission) with (ii) random daily testing.
Results: In the baseline scenario, randomly introducing a silent SARS-CoV-2 infection into a 170-bed LTCF led to large outbreaks, with a cumulative 86 (95% uncertainty interval 6-224) infections after 3 weeks of unmitigated transmission. Efficacy of symptom-based screening was limited by lags to symptom onset and silent asymptomatic and pre-symptomatic transmission. Across scenarios, testing upon admission detected just 34-66% of patients infected upon LTCF entry, and also missed potential introductions from staff. Random daily testing was more effective when _targeting patients than staff, but was overall an inefficient use of limited resources. At high testing capacity (> 10 tests/100 beds/day), cascades were most effective, with a 19-36% probability of detecting outbreaks prior to any nosocomial transmission, and 26-46% prior to first onset of COVID-19 symptoms. Conversely, at low capacity (< 2 tests/100 beds/day), group testing strategies detected outbreaks earliest. Pooling randomly selected patients in a daily group test was most likely to detect outbreaks prior to first symptom onset (16-27%), while pooling patients and staff expressing any COVID-like symptoms was the most efficient means to improve surveillance given resource limitations, compared to the reference requiring only 6-9 additional tests and 11-28 additional swabs to detect outbreaks 1-6 days earlier, prior to an additional 11-22 infections.
Conclusions: COVID-19 surveillance is challenged by delayed or absent clinical symptoms and imperfect diagnostic sensitivity of standard RT-PCR tests. In our analysis, group testing was the most effective and efficient COVID-19 surveillance strategy for resource-limited LTCFs. Testing cascades were even more effective given ample testing resources. Increasing testing capacity and updating surveillance protocols accordingly could facilitate earlier detection of emerging outbreaks, informing a need for urgent intervention in settings with ongoing nosocomial transmission.
Keywords: COVID-19; Computational modelling; Contact network; Infectious disease surveillance; Long-term care; Mathematical modelling; Public health; SARS-CoV-2; Testing; Transmission dynamics.
Conflict of interest statement
LO reports grants from Pfizer, outside the submitted work. All other authors report no competing interests.
Figures
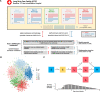
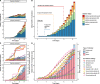
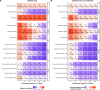
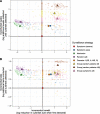
Similar articles
-
Universal screening for SARS-CoV-2 infection: a rapid review.Cochrane Database Syst Rev. 2020 Sep 15;9(9):CD013718. doi: 10.1002/14651858.CD013718. Cochrane Database Syst Rev. 2020. PMID: 33502003 Free PMC article.
-
Early Adoption of Longitudinal Surveillance for SARS-CoV-2 among Staff in Long-Term Care Facilities: Prevalence, Virologic and Sequence Analysis.Microbiol Spectr. 2021 Dec 22;9(3):e0100321. doi: 10.1128/Spectrum.01003-21. Epub 2021 Nov 10. Microbiol Spectr. 2021. PMID: 34756092 Free PMC article.
-
Non-pharmacological measures implemented in the setting of long-term care facilities to prevent SARS-CoV-2 infections and their consequences: a rapid review.Cochrane Database Syst Rev. 2021 Sep 15;9(9):CD015085. doi: 10.1002/14651858.CD015085.pub2. Cochrane Database Syst Rev. 2021. PMID: 34523727 Free PMC article. Review.
-
Effectiveness and cost-effectiveness of four different strategies for SARS-CoV-2 surveillance in the general population (CoV-Surv Study): a structured summary of a study protocol for a cluster-randomised, two-factorial controlled trial.Trials. 2021 Jan 8;22(1):39. doi: 10.1186/s13063-020-04982-z. Trials. 2021. PMID: 33419461 Free PMC article.
-
Rapid, point-of-care antigen tests for diagnosis of SARS-CoV-2 infection.Cochrane Database Syst Rev. 2022 Jul 22;7(7):CD013705. doi: 10.1002/14651858.CD013705.pub3. Cochrane Database Syst Rev. 2022. PMID: 35866452 Free PMC article. Review.
Cited by
-
Evaluating pooled testing for asymptomatic screening of healthcare workers in hospitals.BMC Infect Dis. 2023 Dec 21;23(1):900. doi: 10.1186/s12879-023-08881-x. BMC Infect Dis. 2023. PMID: 38129789 Free PMC article.
-
Impact of non-pharmaceutical interventions on SARS-CoV-2 outbreaks in English care homes: a modelling study.BMC Infect Dis. 2022 Apr 1;22(1):324. doi: 10.1186/s12879-022-07268-8. BMC Infect Dis. 2022. PMID: 35365070 Free PMC article.
-
Impact of contact data resolution on the evaluation of interventions in mathematical models of infectious diseases.J R Soc Interface. 2022 Jun;19(191):20220164. doi: 10.1098/rsif.2022.0164. Epub 2022 Jun 22. J R Soc Interface. 2022. PMID: 35730172 Free PMC article.
-
Identifying optimal COVID-19 testing strategies for schools and businesses: Balancing testing frequency, individual test technology, and cost.PLoS One. 2021 Mar 25;16(3):e0248783. doi: 10.1371/journal.pone.0248783. eCollection 2021. PLoS One. 2021. PMID: 33764982 Free PMC article.
-
Organizational Support Experiences of Care Home and Home Care Staff in Sweden, Italy, Germany and the United Kingdom during the COVID-19 Pandemic.Healthcare (Basel). 2021 Jun 19;9(6):767. doi: 10.3390/healthcare9060767. Healthcare (Basel). 2021. PMID: 34205430 Free PMC article.
References
-
- World Health Organization . Preventing and managing COVID-19 across long-term care services: policy brief. Geneva: World Health Organization; 2020.
-
- Salcher-Konrad M, Jhass A, Naci H, Tan M, El-Tawil Y, Comas-Herrera A. COVID-19 related mortality and spread of disease in long-term care: a living systematic review of emerging evidence. medRxiv. 2020. 10.1101/2020.06.09.20125237.
Publication types
MeSH terms
Grants and funding
LinkOut - more resources
Full Text Sources
Medical
Miscellaneous