Evaluating the Viability of a Smartphone-Based Annotation Tool for Faster and Accurate Image Labelling for Artificial Intelligence in Diabetic Retinopathy
- PMID: 33727785
- PMCID: PMC7953891
- DOI: 10.2147/OPTH.S289425
Evaluating the Viability of a Smartphone-Based Annotation Tool for Faster and Accurate Image Labelling for Artificial Intelligence in Diabetic Retinopathy
Erratum in
-
Erratum: Evaluating the Viability of a Smartphone-Based Annotation Tool for Faster and Accurate Image Labelling for Artificial Intelligence in Diabetic Retinopathy [Erratum].Clin Ophthalmol. 2021 Jun 16;15:2497. doi: 10.2147/OPTH.S324359. eCollection 2021. Clin Ophthalmol. 2021. PMID: 34163133 Free PMC article.
Abstract
Introduction: Deep Learning (DL) and Artificial Intelligence (AI) have become widespread due to the advanced technologies and availability of digital data. Supervised learning algorithms have shown human-level performance or even better and are better feature extractor-quantifier than unsupervised learning algorithms. To get huge dataset with good quality control, there is a need of an annotation tool with a customizable feature set. This paper evaluates the viability of having an in house annotation tool which works on a smartphone and can be used in a healthcare setting.
Methods: We developed a smartphone-based grading system to help researchers in grading multiple retinal fundi. The process consisted of designing the flow of user interface (UI) keeping in view feedback from experts. Quantitative and qualitative analysis of change in speed of a grader over time and feature usage statistics was done. The dataset size was approximately 16,000 images with adjudicated labels by a minimum of 2 doctors. Results for an AI model trained on the images graded using this tool and its validation over some public datasets were prepared.
Results: We created a DL model and analysed its performance for a binary referrable DR Classification task, whether a retinal image has Referrable DR or not. A total of 32 doctors used the tool for minimum of 20 images each. Data analytics suggested significant portability and flexibility of the tool. Grader variability for images was in favour of agreement on images annotated. Number of images used to assess agreement is 550. Mean of 75.9% was seen in agreement.
Conclusion: Our aim was to make Annotation of Medical imaging easier and to minimize time taken for annotations without quality degradation. The user feedback and feature usage statistics confirm our hypotheses of incorporation of brightness and contrast variations, green channels and zooming add-ons in correlation to certain disease types. Simulation of multiple review cycles and establishing quality control can boost the accuracy of AI models even further. Although our study aims at developing an annotation tool for diagnosing and classifying diabetic retinopathy fundus images but same concept can be used for fundus images of other ocular diseases as well as other streams of medical science such as radiology where image-based diagnostic applications are utilised.
Keywords: artificial intelligence; deep learning; referrable diabetic retinopathy.
© 2021 Morya et al.
Conflict of interest statement
The authors report no conflicts of interest in this work.
Figures
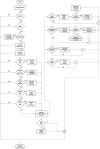
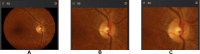
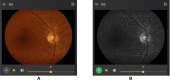
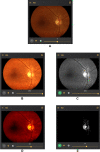
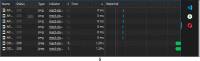
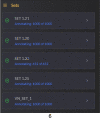
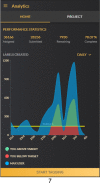
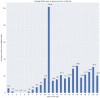
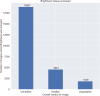
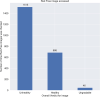
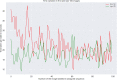
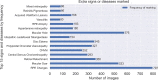
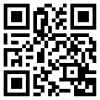
Similar articles
-
Medios- An offline, smartphone-based artificial intelligence algorithm for the diagnosis of diabetic retinopathy.Indian J Ophthalmol. 2020 Feb;68(2):391-395. doi: 10.4103/ijo.IJO_1203_19. Indian J Ophthalmol. 2020. PMID: 31957735 Free PMC article.
-
Validation of Deep Convolutional Neural Network-based algorithm for detection of diabetic retinopathy - Artificial intelligence versus clinician for screening.Indian J Ophthalmol. 2020 Feb;68(2):398-405. doi: 10.4103/ijo.IJO_966_19. Indian J Ophthalmol. 2020. PMID: 31957737 Free PMC article.
-
Diagnostic Accuracy of Community-Based Diabetic Retinopathy Screening With an Offline Artificial Intelligence System on a Smartphone.JAMA Ophthalmol. 2019 Oct 1;137(10):1182-1188. doi: 10.1001/jamaophthalmol.2019.2923. JAMA Ophthalmol. 2019. PMID: 31393538 Free PMC article.
-
RIL-Contour: a Medical Imaging Dataset Annotation Tool for and with Deep Learning.J Digit Imaging. 2019 Aug;32(4):571-581. doi: 10.1007/s10278-019-00232-0. J Digit Imaging. 2019. PMID: 31089974 Free PMC article. Review.
-
The Role of Telemedicine, In-Home Testing and Artificial Intelligence to Alleviate an Increasingly Burdened Healthcare System: Diabetic Retinopathy.Ophthalmol Ther. 2021 Sep;10(3):445-464. doi: 10.1007/s40123-021-00353-2. Epub 2021 Jun 22. Ophthalmol Ther. 2021. PMID: 34156632 Free PMC article. Review.
Cited by
-
Everything real about unreal artificial intelligence in diabetic retinopathy and in ocular pathologies.World J Diabetes. 2022 Oct 15;13(10):822-834. doi: 10.4239/wjd.v13.i10.822. World J Diabetes. 2022. PMID: 36311999 Free PMC article. Review.
-
Active Learning Performance in Labeling Radiology Images Is 90% Effective.Front Radiol. 2021 Nov 30;1:748968. doi: 10.3389/fradi.2021.748968. eCollection 2021. Front Radiol. 2021. PMID: 37492167 Free PMC article.
References
-
- Krizhevsky A, Sutskever I, Hinton: G. ImageNet classification with deep convolutional neural networks. NPIS. 2012;1:1097–1105.
-
- LeCun Y, Kavukcuoglu K, Farabet C. Convolutional networks and applications in vision. Proceedings of 2010 IEEE International Symposium on Circuits and Systems; 2010; Paris: 253–256.
-
- Russakovsky O, Deng J, Su H, et al. ImageNet large scale visual recognition challenge. Int J Comput Vis. 2015;115(3):211–252. doi:10.1007/s11263-015-0816-y - DOI
-
- AI system is better than human doctors at predicting breast cancer. Available from: https://www.newscientist.com/article/2228752-ai-system-is-better-than-hu.... Accessed February12, 2021.
-
- Halevy A, Norvig P, Pereira F. The unreasonable effectiveness of data. IEEE Intell Syst. 2009;24(2):8–12. doi:10.1109/MIS.2009.36 - DOI
LinkOut - more resources
Full Text Sources
Other Literature Sources