Clinically-Driven Virtual Patient Cohorts Generation: An Application to Aorta
- PMID: 34539438
- PMCID: PMC8440937
- DOI: 10.3389/fphys.2021.713118
Clinically-Driven Virtual Patient Cohorts Generation: An Application to Aorta
Abstract
The combination of machine learning methods together with computational modeling and simulation of the cardiovascular system brings the possibility of obtaining very valuable information about new therapies or clinical devices through in-silico experiments. However, the application of machine learning methods demands access to large cohorts of patients. As an alternative to medical data acquisition and processing, which often requires some degree of manual intervention, the generation of virtual cohorts made of synthetic patients can be automated. However, the generation of a synthetic sample can still be computationally demanding to guarantee that it is clinically meaningful and that it reflects enough inter-patient variability. This paper addresses the problem of generating virtual patient cohorts of thoracic aorta geometries that can be used for in-silico trials. In particular, we focus on the problem of generating a cohort of patients that meet a particular clinical criterion, regardless the access to a reference sample of that phenotype. We formalize the problem of clinically-driven sampling and assess several sampling strategies with two goals, sampling efficiency, i.e., that the generated individuals actually belong to the _target population, and that the statistical properties of the cohort can be controlled. Our results show that generative adversarial networks can produce reliable, clinically-driven cohorts of thoracic aortas with good efficiency. Moreover, non-linear predictors can serve as an efficient alternative to the sometimes expensive evaluation of anatomical or functional parameters of the organ of interest.
Keywords: clinically-driven sampling; digital twin; generative adversarial network; in-silico trials; support vector machine; synthetic population; thoracic-aorta; virtual cohort.
Copyright © 2021 Romero, Lozano, Martínez-Gil, Serra, Sebastián, Lamata and García-Fernández.
Conflict of interest statement
The authors declare that the research was conducted in the absence of any commercial or financial relationships that could be construed as a potential conflict of interest.
Figures
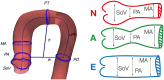
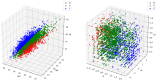
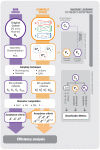
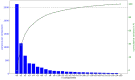
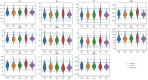
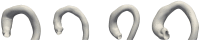
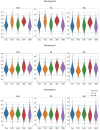
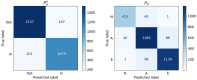
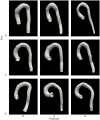
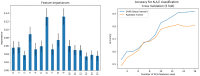
Similar articles
-
Generation of synthetic aortic valve stenosis geometries for in silico trials.Int J Numer Method Biomed Eng. 2024 Jan;40(1):e3778. doi: 10.1002/cnm.3778. Epub 2023 Nov 14. Int J Numer Method Biomed Eng. 2024. PMID: 37961993
-
A method for machine learning generation of realistic synthetic datasets for validating healthcare applications.Health Informatics J. 2022 Apr-Jun;28(2):14604582221077000. doi: 10.1177/14604582221077000. Health Informatics J. 2022. PMID: 35414269
-
Generating synthetic personal health data using conditional generative adversarial networks combining with differential privacy.J Biomed Inform. 2023 Jul;143:104404. doi: 10.1016/j.jbi.2023.104404. Epub 2023 Jun 1. J Biomed Inform. 2023. PMID: 37268168
-
Synthetic biomedical data generation in support of In Silico Clinical Trials.Front Big Data. 2023 Aug 15;6:1085571. doi: 10.3389/fdata.2023.1085571. eCollection 2023. Front Big Data. 2023. PMID: 37655113 Free PMC article. Review.
-
Data-driven modeling and prediction of blood glucose dynamics: Machine learning applications in type 1 diabetes.Artif Intell Med. 2019 Jul;98:109-134. doi: 10.1016/j.artmed.2019.07.007. Epub 2019 Jul 26. Artif Intell Med. 2019. PMID: 31383477 Review.
Cited by
-
Interpretable cardiac anatomy modeling using variational mesh autoencoders.Front Cardiovasc Med. 2022 Dec 22;9:983868. doi: 10.3389/fcvm.2022.983868. eCollection 2022. Front Cardiovasc Med. 2022. PMID: 36620629 Free PMC article.
-
Simulating impaired left ventricular-arterial coupling in aging and disease: a systematic review.Biomed Eng Online. 2024 Feb 22;23(1):24. doi: 10.1186/s12938-024-01206-2. Biomed Eng Online. 2024. PMID: 38388416 Free PMC article.
-
Training machine learning models with synthetic data improves the prediction of ventricular origin in outflow tract ventricular arrhythmias.Front Physiol. 2022 Aug 12;13:909372. doi: 10.3389/fphys.2022.909372. eCollection 2022. Front Physiol. 2022. PMID: 36035489 Free PMC article.
-
Multi-Domain Variational Autoencoders for Combined Modeling of MRI-Based Biventricular Anatomy and ECG-Based Cardiac Electrophysiology.Front Physiol. 2022 Jun 8;13:886723. doi: 10.3389/fphys.2022.886723. eCollection 2022. Front Physiol. 2022. PMID: 35755443 Free PMC article.
-
Using machine learning surrogate modeling for faster QSP VP cohort generation.CPT Pharmacometrics Syst Pharmacol. 2023 Aug;12(8):1047-1059. doi: 10.1002/psp4.12999. Epub 2023 Jun 16. CPT Pharmacometrics Syst Pharmacol. 2023. PMID: 37328956 Free PMC article.
References
-
- Amidan B., Ferryman T., Cooley S. (2005). “Data outlier detection using the Chebyshev theorem,” in 2005 IEEE Aerospace Conference (Big Sky, MT: IEEE; ), 3814–3819.
-
- Bishop C. M. (2006). Pattern Recognition and Machine Learning (Information Science and Statistics). Berlin; Heidelberg: Springer-Verlag.
-
- Bratt A., Kim J., Pollie M., Beecy A. N., Tehrani N. H., Codella N., et al. . (2019). Machine learning derived segmentation of phase velocity encoded cardiovascular magnetic resonance for fully automated aortic flow quantification. J. Cardiovasc. Magn. Reson. 21, 1. 10.1186/s12968-018-0509-0 - DOI - PMC - PubMed
-
- Britton O. J., Bueno-Orovio A., Van Ammel K., Lu H. R., Towart R., Gallacher D. J., et al. . (2013). Experimentally calibrated population of models predicts and explains intersubject variability in cardiac cellular electrophysiology. Proc. Natl. Acad. Sci. U.S.A. 110, E2098–E2105. 10.1073/pnas.1304382110 - DOI - PMC - PubMed
Grants and funding
LinkOut - more resources
Full Text Sources
Research Materials