Unsupervised domain adaptation based COVID-19 CT infection segmentation network
- PMID: 34764618
- PMCID: PMC8421243
- DOI: 10.1007/s10489-021-02691-x
Unsupervised domain adaptation based COVID-19 CT infection segmentation network
Abstract
Automatic segmentation of infection areas in computed tomography (CT) images has proven to be an effective diagnostic approach for COVID-19. However, due to the limited number of pixel-level annotated medical images, accurate segmentation remains a major challenge. In this paper, we propose an unsupervised domain adaptation based segmentation network to improve the segmentation performance of the infection areas in COVID-19 CT images. In particular, we propose to utilize the synthetic data and limited unlabeled real COVID-19 CT images to jointly train the segmentation network. Furthermore, we develop a novel domain adaptation module, which is used to align the two domains and effectively improve the segmentation network's generalization capability to the real domain. Besides, we propose an unsupervised adversarial training scheme, which encourages the segmentation network to learn the domain-invariant feature, so that the robust feature can be used for segmentation. Experimental results demonstrate that our method can achieve state-of-the-art segmentation performance on COVID-19 CT images.
Keywords: Adversarial training; Automatic segmentation; COVID-19; Computed tomography; Domain adaptation.
© The Author(s), under exclusive licence to Springer Science+Business Media, LLC, part of Springer Nature 2021.
Figures
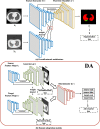
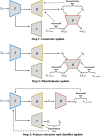
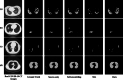
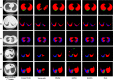
Similar articles
-
Multiscale unsupervised domain adaptation for automatic pancreas segmentation in CT volumes using adversarial learning.Med Phys. 2022 Sep;49(9):5799-5818. doi: 10.1002/mp.15827. Epub 2022 Jul 27. Med Phys. 2022. PMID: 35833617
-
A teacher-student framework with Fourier Transform augmentation for COVID-19 infection segmentation in CT images.Biomed Signal Process Control. 2023 Jan;79:104250. doi: 10.1016/j.bspc.2022.104250. Epub 2022 Sep 26. Biomed Signal Process Control. 2023. PMID: 36188130 Free PMC article.
-
Two-stage adversarial learning based unsupervised domain adaptation for retinal OCT segmentation.Med Phys. 2024 Aug;51(8):5374-5385. doi: 10.1002/mp.17012. Epub 2024 Mar 1. Med Phys. 2024. PMID: 38426594
-
Bidirectional cross-modality unsupervised domain adaptation using generative adversarial networks for cardiac image segmentation.Comput Biol Med. 2021 Sep;136:104726. doi: 10.1016/j.compbiomed.2021.104726. Epub 2021 Aug 4. Comput Biol Med. 2021. PMID: 34371318
-
ECSD-Net: A joint optic disc and cup segmentation and glaucoma classification network based on unsupervised domain adaptation.Comput Methods Programs Biomed. 2022 Jan;213:106530. doi: 10.1016/j.cmpb.2021.106530. Epub 2021 Nov 14. Comput Methods Programs Biomed. 2022. PMID: 34813984 Review.
Cited by
-
The Advent of Domain Adaptation into Artificial Intelligence for Gastrointestinal Endoscopy and Medical Imaging.Diagnostics (Basel). 2023 Sep 22;13(19):3023. doi: 10.3390/diagnostics13193023. Diagnostics (Basel). 2023. PMID: 37835766 Free PMC article. Review.
-
Supervised and weakly supervised deep learning models for COVID-19 CT diagnosis: A systematic review.Comput Methods Programs Biomed. 2022 May;218:106731. doi: 10.1016/j.cmpb.2022.106731. Epub 2022 Mar 5. Comput Methods Programs Biomed. 2022. PMID: 35286874 Free PMC article. Review.
-
A fast lightweight network for the discrimination of COVID-19 and pulmonary diseases.Biomed Signal Process Control. 2022 Sep;78:103925. doi: 10.1016/j.bspc.2022.103925. Epub 2022 Jun 21. Biomed Signal Process Control. 2022. PMID: 35755317 Free PMC article.
-
Association between emphysema and other pulmonary computed tomography patterns in COVID-19 pneumonia.J Med Virol. 2023 Jan;95(1):e28293. doi: 10.1002/jmv.28293. Epub 2022 Nov 17. J Med Virol. 2023. PMID: 36358023 Free PMC article.
-
A radiological image analysis framework for early screening of the COVID-19 infection: A computer vision-based approach.Appl Soft Comput. 2022 Apr;119:108528. doi: 10.1016/j.asoc.2022.108528. Epub 2022 Feb 3. Appl Soft Comput. 2022. PMID: 35136390 Free PMC article.
References
-
- Ortiz-Ospina E, Ritchie H et al (2021) Mortality risk of covid-19. https://ourworldindata.org/mortality-risk-covid
-
- Gozes O, Frid-Adar M, Greenspan H, Browning PD, Zhang H, Ji W, Adam B, Eliot S (2020) Rapid ai development cycle for the coronavirus (covid-19) pandemic: Initial results for automated detection & patient monitoring using deep learning ct image analysis. arXiv:2003.05037
-
- Shan F, Gao Y, Wang J, Shi W, Shi N, Han M, Xue Z, Shi Y (2020) Lung infection quantification of covid-19 in ct images with deep learning. arXiv:2003.04655
LinkOut - more resources
Full Text Sources