Strategies to Increase Prediction Accuracy in Genomic Selection of Complex Traits in Alfalfa (Medicago sativa L.)
- PMID: 34943880
- PMCID: PMC8699225
- DOI: 10.3390/cells10123372
Strategies to Increase Prediction Accuracy in Genomic Selection of Complex Traits in Alfalfa (Medicago sativa L.)
Abstract
Agronomic traits such as biomass yield and abiotic stress tolerance are genetically complex and challenging to improve through conventional breeding approaches. Genomic selection (GS) is an alternative approach in which genome-wide markers are used to determine the genomic estimated breeding value (GEBV) of individuals in a population. In alfalfa (Medicago sativa L.), previous results indicated that low to moderate prediction accuracy values (<70%) were obtained in complex traits, such as yield and abiotic stress resistance. There is a need to increase the prediction value in order to employ GS in breeding programs. In this paper we reviewed different statistic models and their applications in polyploid crops, such as alfalfa and potato. Specifically, we used empirical data affiliated with alfalfa yield under salt stress to investigate approaches that use DNA marker importance values derived from machine learning models, and genome-wide association studies (GWAS) of marker-trait association scores based on different GWASpoly models, in weighted GBLUP analyses. This approach increased prediction accuracies from 50% to more than 80% for alfalfa yield under salt stress. Finally, we expended the weighted GBLUP approach to potato and analyzed 13 phenotypic traits and obtained similar results. This is the first report on alfalfa to use variable importance and GWAS-assisted approaches to increase the prediction accuracy of GS, thus helping to select superior alfalfa lines based on their GEBVs.
Keywords: Medicago sativa; WGBLUP; genomic selection.
Conflict of interest statement
The authors do not have conflict of interest.
Figures
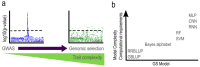
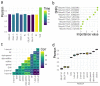
Similar articles
-
Genome-Wide Association and Prediction of Traits Related to Salt Tolerance in Autotetraploid Alfalfa (Medicago sativa L.).Int J Mol Sci. 2020 May 9;21(9):3361. doi: 10.3390/ijms21093361. Int J Mol Sci. 2020. PMID: 32397526 Free PMC article.
-
Genome-Wide Association Mapping and Genomic Selection for Alfalfa (Medicago sativa) Forage Quality Traits.PLoS One. 2017 Jan 9;12(1):e0169234. doi: 10.1371/journal.pone.0169234. eCollection 2017. PLoS One. 2017. PMID: 28068350 Free PMC article.
-
Genomic Prediction for 25 Agronomic and Quality Traits in Alfalfa (Medicago sativa).Front Plant Sci. 2018 Aug 20;9:1220. doi: 10.3389/fpls.2018.01220. eCollection 2018. Front Plant Sci. 2018. PMID: 30177947 Free PMC article.
-
A stepwise guide for pangenome development in crop plants: an alfalfa (Medicago sativa) case study.BMC Genomics. 2024 Oct 31;25(1):1022. doi: 10.1186/s12864-024-10931-w. BMC Genomics. 2024. PMID: 39482604 Free PMC article. Review.
-
Biotechnological Perspectives of Omics and Genetic Engineering Methods in Alfalfa.Front Plant Sci. 2020 May 21;11:592. doi: 10.3389/fpls.2020.00592. eCollection 2020. Front Plant Sci. 2020. PMID: 32508859 Free PMC article. Review.
Cited by
-
Application of machine learning to explore the genomic prediction accuracy of fall dormancy in autotetraploid alfalfa.Hortic Res. 2022 Oct 7;10(1):uhac225. doi: 10.1093/hr/uhac225. eCollection 2023. Hortic Res. 2022. PMID: 36643744 Free PMC article.
-
Genome-wide family prediction unveils molecular mechanisms underlying the regulation of agronomic traits in Urochloa ruziziensis.Front Plant Sci. 2023 Dec 12;14:1303417. doi: 10.3389/fpls.2023.1303417. eCollection 2023. Front Plant Sci. 2023. PMID: 38148869 Free PMC article.
-
Combining GS-assisted GWAS and transcriptome analysis to mine candidate genes for nitrogen utilization efficiency in Populus cathayana.BMC Plant Biol. 2023 Apr 5;23(1):182. doi: 10.1186/s12870-023-04202-1. BMC Plant Biol. 2023. PMID: 37020197 Free PMC article.
-
Alfalfa Mosaic Virus and White Clover Mosaic Virus Combined Infection Leads to Chloroplast Destruction and Alterations in Photosynthetic Characteristics of Nicotiana benthamiana.Viruses. 2024 Aug 5;16(8):1255. doi: 10.3390/v16081255. Viruses. 2024. PMID: 39205229 Free PMC article.
-
Genome-wide genotyping data renew knowledge on genetic diversity of a worldwide alfalfa collection and give insights on genetic control of phenology traits.Front Plant Sci. 2023 Jul 5;14:1196134. doi: 10.3389/fpls.2023.1196134. eCollection 2023. Front Plant Sci. 2023. PMID: 37476178 Free PMC article.
References
Publication types
MeSH terms
Grants and funding
LinkOut - more resources
Full Text Sources