Exploring Silent Speech Interfaces Based on Frequency-Modulated Continuous-Wave Radar
- PMID: 35062610
- PMCID: PMC8781659
- DOI: 10.3390/s22020649
Exploring Silent Speech Interfaces Based on Frequency-Modulated Continuous-Wave Radar
Abstract
Speech is our most natural and efficient form of communication and offers a strong potential to improve how we interact with machines. However, speech communication can sometimes be limited by environmental (e.g., ambient noise), contextual (e.g., need for privacy), or health conditions (e.g., laryngectomy), preventing the consideration of audible speech. In this regard, silent speech interfaces (SSI) have been proposed as an alternative, considering technologies that do not require the production of acoustic signals (e.g., electromyography and video). Unfortunately, despite their plentitude, many still face limitations regarding their everyday use, e.g., being intrusive, non-portable, or raising technical (e.g., lighting conditions for video) or privacy concerns. In line with this necessity, this article explores the consideration of contactless continuous-wave radar to assess its potential for SSI development. A corpus of 13 European Portuguese words was acquired for four speakers and three of them enrolled in a second acquisition session, three months later. Regarding the speaker-dependent models, trained and tested with data from each speaker while using 5-fold cross-validation, average accuracies of 84.50% and 88.00% were respectively obtained from Bagging (BAG) and Linear Regression (LR) classifiers, respectively. Additionally, recognition accuracies of 81.79% and 81.80% were also, respectively, achieved for the session and speaker-independent experiments, establishing promising grounds for further exploring this technology towards silent speech recognition.
Keywords: European Portuguese; continuous-wave radar; machine learning; silent speech.
Conflict of interest statement
The authors declare no conflict of interest. The funders had no role in the design of the study; in the collection, analyses, or interpretation of data; in the writing of the manuscript, or in the decision to publish the results.
Figures

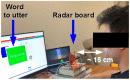
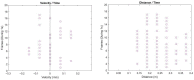
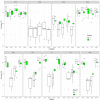
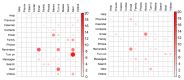
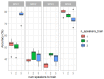
Similar articles
-
Silent speech command word recognition using stepped frequency continuous wave radar.Sci Rep. 2022 Mar 9;12(1):4192. doi: 10.1038/s41598-022-07842-9. Sci Rep. 2022. PMID: 35273225 Free PMC article.
-
Towards Contactless Silent Speech Recognition Based on Detection of Active and Visible Articulators Using IR-UWB Radar.Sensors (Basel). 2016 Oct 29;16(11):1812. doi: 10.3390/s16111812. Sensors (Basel). 2016. PMID: 27801867 Free PMC article.
-
Development of sEMG sensors and algorithms for silent speech recognition.J Neural Eng. 2018 Aug;15(4):046031. doi: 10.1088/1741-2552/aac965. Epub 2018 Jun 1. J Neural Eng. 2018. PMID: 29855428 Free PMC article.
-
Application of Deep Learning on Millimeter-Wave Radar Signals: A Review.Sensors (Basel). 2021 Mar 10;21(6):1951. doi: 10.3390/s21061951. Sensors (Basel). 2021. PMID: 33802217 Free PMC article. Review.
-
Noise and communication: a three-year update.Noise Health. 2012 Nov-Dec;14(61):281-6. doi: 10.4103/1463-1741.104894. Noise Health. 2012. PMID: 23257578 Review.
Cited by
-
A data-efficient and easy-to-use lip language interface based on wearable motion capture and speech movement reconstruction.Sci Adv. 2024 Jun 28;10(26):eado9576. doi: 10.1126/sciadv.ado9576. Epub 2024 Jun 26. Sci Adv. 2024. PMID: 38924408 Free PMC article.
-
Speaker Adaptation on Articulation and Acoustics for Articulation-to-Speech Synthesis.Sensors (Basel). 2022 Aug 13;22(16):6056. doi: 10.3390/s22166056. Sensors (Basel). 2022. PMID: 36015817 Free PMC article.
-
Optimizing the Ultrasound Tongue Image Representation for Residual Network-Based Articulatory-to-Acoustic Mapping.Sensors (Basel). 2022 Nov 8;22(22):8601. doi: 10.3390/s22228601. Sensors (Basel). 2022. PMID: 36433196 Free PMC article.
-
A comprehensive multimodal dataset for contactless lip reading and acoustic analysis.Sci Data. 2023 Dec 13;10(1):895. doi: 10.1038/s41597-023-02793-w. Sci Data. 2023. PMID: 38092796 Free PMC article.
References
-
- Kepuska V., Bohouta G. Next-generation of virtual personal assistants (Microsoft Cortana, Apple Siri, Amazon Alexa and Google Home); Proceedings of the 2018 IEEE 8th Annual Computing and Communication Workshop and Conference (CCWC); Las Vegas, NV, USA. 8–10 January 2018; pp. 99–103.
-
- Denby B., Schultz T., Honda K., Hueber T., Gilbert J.M., Brumberg J.S. Silent speech interfaces. Speech Commun. 2010;52:270–287. doi: 10.1016/j.specom.2009.08.002. - DOI
-
- Levelt W.J. Speaking: From Intention to Articulation. Volume 1 MIT Press; Cambridge, MA, USA: 1993.
-
- Freitas J., Teixeira A., Dias M.S., Silva S. An Introduction to Silent Speech Interfaces. Springer; Berlin/Heidelberg, Germany: 2017. SSI Modalities I: Behind the Scenes—From the Brain to the Muscles; pp. 15–30.
MeSH terms
LinkOut - more resources
Full Text Sources