Multi-ancestry genetic study of type 2 diabetes highlights the power of diverse populations for discovery and translation
- PMID: 35551307
- PMCID: PMC9179018
- DOI: 10.1038/s41588-022-01058-3
Multi-ancestry genetic study of type 2 diabetes highlights the power of diverse populations for discovery and translation
Abstract
We assembled an ancestrally diverse collection of genome-wide association studies (GWAS) of type 2 diabetes (T2D) in 180,834 affected individuals and 1,159,055 controls (48.9% non-European descent) through the Diabetes Meta-Analysis of Trans-Ethnic association studies (DIAMANTE) Consortium. Multi-ancestry GWAS meta-analysis identified 237 loci attaining stringent genome-wide significance (P < 5 × 10-9), which were delineated to 338 distinct association signals. Fine-mapping of these signals was enhanced by the increased sample size and expanded population diversity of the multi-ancestry meta-analysis, which localized 54.4% of T2D associations to a single variant with >50% posterior probability. This improved fine-mapping enabled systematic assessment of candidate causal genes and molecular mechanisms through which T2D associations are mediated, laying the foundations for functional investigations. Multi-ancestry genetic risk scores enhanced transferability of T2D prediction across diverse populations. Our study provides a step toward more effective clinical translation of T2D GWAS to improve global health for all, irrespective of genetic background.
© 2022. The Author(s), under exclusive licence to Springer Nature America, Inc.
Figures
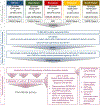
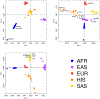
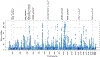
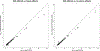
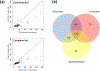
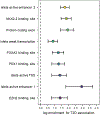
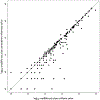
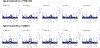
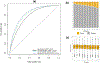
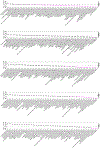
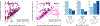
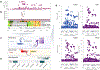
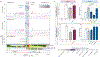
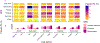
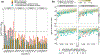
Similar articles
-
A proteome-wide association study identifies putative causal proteins for breast cancer risk.Br J Cancer. 2024 Dec;131(11):1796-1804. doi: 10.1038/s41416-024-02879-1. Epub 2024 Oct 28. Br J Cancer. 2024. PMID: 39468330 Free PMC article.
-
Genedrive kit for detecting single nucleotide polymorphism m.1555A>G in neonates and their mothers: a systematic review and cost-effectiveness analysis.Health Technol Assess. 2024 Oct;28(75):1-75. doi: 10.3310/TGAC4201. Health Technol Assess. 2024. PMID: 39487741 Free PMC article.
-
Depressing time: Waiting, melancholia, and the psychoanalytic practice of care.In: Kirtsoglou E, Simpson B, editors. The Time of Anthropology: Studies of Contemporary Chronopolitics. Abingdon: Routledge; 2020. Chapter 5. In: Kirtsoglou E, Simpson B, editors. The Time of Anthropology: Studies of Contemporary Chronopolitics. Abingdon: Routledge; 2020. Chapter 5. PMID: 36137063 Free Books & Documents. Review.
-
Comparison of Two Modern Survival Prediction Tools, SORG-MLA and METSSS, in Patients With Symptomatic Long-bone Metastases Who Underwent Local Treatment With Surgery Followed by Radiotherapy and With Radiotherapy Alone.Clin Orthop Relat Res. 2024 Dec 1;482(12):2193-2208. doi: 10.1097/CORR.0000000000003185. Epub 2024 Jul 23. Clin Orthop Relat Res. 2024. PMID: 39051924
-
Undernutrition as a risk factor for tuberculosis disease.Cochrane Database Syst Rev. 2024 Jun 11;6(6):CD015890. doi: 10.1002/14651858.CD015890.pub2. Cochrane Database Syst Rev. 2024. PMID: 38860538 Free PMC article. Review.
Cited by
-
Transcriptome-wide Mendelian randomization during CD4+ T cell activation reveals immune-related drug _targets for cardiometabolic diseases.Nat Commun. 2024 Oct 28;15(1):9302. doi: 10.1038/s41467-024-53621-7. Nat Commun. 2024. PMID: 39468075 Free PMC article.
-
The Role of Electronic Health Records in Advancing Genomic Medicine.Annu Rev Genomics Hum Genet. 2021 Aug 31;22:219-238. doi: 10.1146/annurev-genom-121120-125204. Epub 2021 May 26. Annu Rev Genomics Hum Genet. 2021. PMID: 34038146 Free PMC article. Review.
-
Cardiometabolic and renal phenotypes and transitions in the United States population.Nat Cardiovasc Res. 2023 Dec 15;3(1):46-59. doi: 10.1038/s44161-023-00391-y. Nat Cardiovasc Res. 2023. PMID: 38314318 Free PMC article.
-
Predicting Type 2 Diabetes Metabolic Phenotypes Using Continuous Glucose Monitoring and a Machine Learning Framework.medRxiv [Preprint]. 2024 Sep 9:2024.07.20.24310737. doi: 10.1101/2024.07.20.24310737. medRxiv. 2024. PMID: 39108516 Free PMC article. Preprint.
-
Metabolic Stress Levels Influence the Ability of Myelin Transcription Factors to Regulate β-Cell Identity and Survival.Diabetes. 2024 Oct 1;73(10):1662-1672. doi: 10.2337/db23-0528. Diabetes. 2024. PMID: 39058602
References
-
- GBD 2015 Disease and Injury Incidence and Prevalence Collaborators. Global, regional, and national incidence, prevalence, and years lived with disability for 310 diseases and injuries, 1990-2015: a systematic analysis for the Global Burden of Disease Study 2015. Lancet 388, 1545–1602 (2016). - PMC - PubMed
Publication types
MeSH terms
Grants and funding
- 200837 /WT_/Wellcome Trust/United Kingdom
- R01 HL142302/HL/NHLBI NIH HHS/United States
- R35 HL135818/HL/NHLBI NIH HHS/United States
- MC_PC_13049/MRC_/Medical Research Council/United Kingdom
- 203141 /WT_/Wellcome Trust/United Kingdom
- R01 DK039311/DK/NIDDK NIH HHS/United States
- R01 DK093757/DK/NIDDK NIH HHS/United States
- R01 DK078616/DK/NIDDK NIH HHS/United States
- R00 AG066849/AG/NIA NIH HHS/United States
- L30 DK126146/DK/NIDDK NIH HHS/United States
- MC_UU_00006/1/MRC_/Medical Research Council/United Kingdom
- MR/L020149/1/MRC_/Medical Research Council/United Kingdom
- 086113 /WT_/Wellcome Trust/United Kingdom
- 064890 /WT_/Wellcome Trust/United Kingdom
- R01 DK114650/DK/NIDDK NIH HHS/United States
- R03 DK131249/DK/NIDDK NIH HHS/United States
- U01 DK085545/DK/NIDDK NIH HHS/United States
- 212946/Z/18/Z/WT_/Wellcome Trust/United Kingdom
- 088158 /WT_/Wellcome Trust/United Kingdom
- 101630 /WT_/Wellcome Trust/United Kingdom
- 090532 /WT_/Wellcome Trust/United Kingdom
- K99 AG066849/AG/NIA NIH HHS/United States
- K23 DK114551/DK/NIDDK NIH HHS/United States
- 212284 /WT_/Wellcome Trust/United Kingdom
- MC_UU_12026/2/MRC_/Medical Research Council/United Kingdom
- 206194 /WT_/Wellcome Trust/United Kingdom
- R01 DK098032/DK/NIDDK NIH HHS/United States
- 212946 /WT_/Wellcome Trust/United Kingdom
- 212945/Z/18/Z/WT_/Wellcome Trust/United Kingdom
- R01 HL153805/HL/NHLBI NIH HHS/United States
- R01 DK072193/DK/NIDDK NIH HHS/United States
- 212284/Z/18/Z/WT_/Wellcome Trust/United Kingdom
- R01 AG065357/AG/NIA NIH HHS/United States
- 220457 /WT_/Wellcome Trust/United Kingdom
- G0700931/MRC_/Medical Research Council/United Kingdom
- 202922 /WT_/Wellcome Trust/United Kingdom
- 072960 /WT_/Wellcome Trust/United Kingdom
- 104085 /WT_/Wellcome Trust/United Kingdom
- U01 DK105535/DK/NIDDK NIH HHS/United States
- 101033 /WT_/Wellcome Trust/United Kingdom
- R01 DK062370/DK/NIDDK NIH HHS/United States
- R01 HL105756/HL/NHLBI NIH HHS/United States
- P30 ES030285/ES/NIEHS NIH HHS/United States
- 098381 /WT_/Wellcome Trust/United Kingdom
- MC_UU_00017/1/MRC_/Medical Research Council/United Kingdom
- 083948 /WT_/Wellcome Trust/United Kingdom
- 098051 /WT_/Wellcome Trust/United Kingdom
- 212259 /WT_/Wellcome Trust/United Kingdom
- MC_U137686851/MRC_/Medical Research Council/United Kingdom
- R01 DK118011/DK/NIDDK NIH HHS/United States
- P30 DK020541/DK/NIDDK NIH HHS/United States
- MR/R010676/1/MRC_/Medical Research Council/United Kingdom
- U01 DK078616/DK/NIDDK NIH HHS/United States
- U24 AG051129/AG/NIA NIH HHS/United States
- U01 DK062370/DK/NIDDK NIH HHS/United States
- R01 DK105588/DK/NIDDK NIH HHS/United States
- 085475 /WT_/Wellcome Trust/United Kingdom
- MR/L02036X/1/MRC_/Medical Research Council/United Kingdom
- 200186 /WT_/Wellcome Trust/United Kingdom
- G0601966/MRC_/Medical Research Council/United Kingdom
- R01 DK090111/DK/NIDDK NIH HHS/United States
- 095101 /WT_/Wellcome Trust/United Kingdom
- 090367 /WT_/Wellcome Trust/United Kingdom
- UL1 TR001855/TR/NCATS NIH HHS/United States
- R03 HL154284/HL/NHLBI NIH HHS/United States
- WT_/Wellcome Trust/United Kingdom
- UM1 DK078616/DK/NIDDK NIH HHS/United States
- MR/S019669/1/MRC_/Medical Research Council/United Kingdom
- MC_PC_14135/MRC_/Medical Research Council/United Kingdom
- 200837/Z/16/Z/WT_/Wellcome Trust/United Kingdom
- 084723 /WT_/Wellcome Trust/United Kingdom
- 106130 /WT_/Wellcome Trust/United Kingdom
- 098017 /WT_/Wellcome Trust/United Kingdom
- 098395 /WT_/Wellcome Trust/United Kingdom