Cuproptosis-related gene signature stratifies lower-grade glioma patients and predicts immune characteristics
- PMID: 36386799
- PMCID: PMC9640744
- DOI: 10.3389/fgene.2022.1036460
Cuproptosis-related gene signature stratifies lower-grade glioma patients and predicts immune characteristics
Abstract
Cuproptosis is the most recently discovered type of regulated cell death and is mediated by copper ions. Studies show that cuproptosis plays a significant role in cancer development and progression. Lower-grade gliomas (LGGs) are slow-growing brain tumors. The majority of LGGs progress to high-grade glioma, which makes it difficult to predict the prognosis. However, the prognostic value of cuproptosis-related genes (CRGs) in LGG needs to be further explored. mRNA expression profiles and clinical data of LGG patients were collected from public sources for this study. Univariate Cox regression analysis and the least absolute shrinkage and selection operator (LASSO) Cox regression model were used to build a multigene signature that could divide patients into different risk groups. The differences in clinical pathological characteristics, immune infiltration characteristics, and mutation status were evaluated in risk subgroups. In addition, drug sensitivity and immune checkpoint scores were estimated in risk subgroups to provide LGG patients with precision medication. We found that all CRGs were differentially expressed in LGG and normal tissues. Patients were divided into high- and low-risk groups based on the risk score of the CRG signature. Patients in the high-risk group had a considerably lower overall survival rate than those in the low-risk group. According to functional analysis, pathways related to the immune system were enriched, and the immune state differed across the two risk groups. Immune characteristic analysis showed that the immune cell proportion and immune scores were different in the different groups. High-risk group was characterized by low sensitivity to chemotherapy but high sensitivity to immune checkpoint inhibitors. The current study revealed that the novel CRG signature was related to the prognosis, clinicopathological features, immune characteristics, and treatment perference of LGG.
Keywords: cuproptosis-related gene; gene signature; immune score; lower-grade glioma; prognosis.
Copyright © 2022 Zhang, Wang, Xu and Xin.
Figures
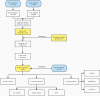
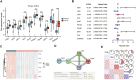
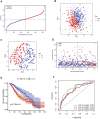
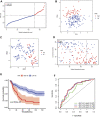
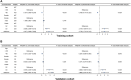
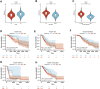
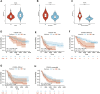
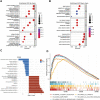
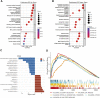
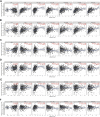
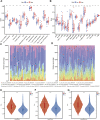
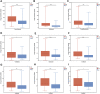
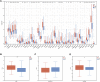
Similar articles
-
Identification of a novel cuproptosis-related gene signature and integrative analyses in patients with lower-grade gliomas.Front Immunol. 2022 Aug 15;13:933973. doi: 10.3389/fimmu.2022.933973. eCollection 2022. Front Immunol. 2022. PMID: 36045691 Free PMC article. Review.
-
Cuproptosis-related gene-located DNA methylation in lower-grade glioma: Prognosis and tumor microenvironment.Cancer Biomark. 2024;40(2):185-198. doi: 10.3233/CBM-230341. Cancer Biomark. 2024. PMID: 38578883 Free PMC article.
-
Development and validation of cuproptosis molecular subtype-related signature for predicting immune prognostic characterization in gliomas.J Cancer Res Clin Oncol. 2023 Oct;149(13):11499-11515. doi: 10.1007/s00432-023-05021-5. Epub 2023 Jul 1. J Cancer Res Clin Oncol. 2023. PMID: 37392200
-
Novel Cuproptosis-Related Gene Signature for Precise Identification of High-Risk Populations in Low-Grade Gliomas.Mediators Inflamm. 2023 Feb 13;2023:6232620. doi: 10.1155/2023/6232620. eCollection 2023. Mediators Inflamm. 2023. PMID: 36814682 Free PMC article.
-
Machine learning algorithm to construct cuproptosis- and immune-related prognosis prediction model for colon cancer.World J Gastrointest Oncol. 2023 Mar 15;15(3):372-388. doi: 10.4251/wjgo.v15.i3.372. World J Gastrointest Oncol. 2023. PMID: 37009317 Free PMC article. Review.
Cited by
-
Machine learning predicts cuproptosis-related lncRNAs and survival in glioma patients.Sci Rep. 2024 Sep 27;14(1):22323. doi: 10.1038/s41598-024-72664-w. Sci Rep. 2024. PMID: 39333603 Free PMC article.
-
Immune-related gene signature to predict TACE refractoriness in patients with hepatocellular carcinoma based on artificial neural network.Front Genet. 2023 Jan 4;13:993509. doi: 10.3389/fgene.2022.993509. eCollection 2022. Front Genet. 2023. PMID: 36685822 Free PMC article.
-
Analysis of cuproptosis-related genes in prognosis and immune infiltration in grade 4 diffuse gliomas.Heliyon. 2024 Apr 9;10(7):e29212. doi: 10.1016/j.heliyon.2024.e29212. eCollection 2024 Apr 15. Heliyon. 2024. PMID: 38633656 Free PMC article.
References
-
- Buccarelli M., D'Alessandris Q. G., Matarrese P., Mollinari C., Signore M., Cappannini A., et al. (2021). Elesclomol-induced increase of mitochondrial reactive oxygen species impairs glioblastoma stem-like cell survival and tumor growth. J. Exp. Clin. Cancer Res. 40 (1), 228. 10.1186/s13046-021-02031-4 - DOI - PMC - PubMed
-
- Cai Z., Zhao J. S., Li J. J., Peng D. N., Wang X. Y., Chen T. L., et al. (2010). A combined proteomics and metabolomics profiling of gastric cardia cancer reveals characteristic dysregulations in glucose metabolism. Mol. Cell. Proteomics 9 (12), 2617–2628. 10.1074/mcp.M110.000661 - DOI - PMC - PubMed
LinkOut - more resources
Full Text Sources