Microbiome Dysbiosis Shows Strong Association of Gut-Derived Altered Metabolomic Profile in Gulf War Chronic Multisymptom Illness Symptom Persistence Following Western Diet Feeding and Development of Obesity
- PMID: 36835663
- PMCID: PMC9962797
- DOI: 10.3390/ijms24044245
Microbiome Dysbiosis Shows Strong Association of Gut-Derived Altered Metabolomic Profile in Gulf War Chronic Multisymptom Illness Symptom Persistence Following Western Diet Feeding and Development of Obesity
Abstract
The pathophysiology of Gulf War Illness (GWI) remains elusive even after three decades. The persistence of multiple complex symptoms along with metabolic disorders such as obesity worsens the health of present Gulf War (GW) Veterans often by the interactions of the host gut microbiome and inflammatory mediators. In this study, we hypothesized that the administration of a Western diet might alter the host metabolomic profile, which is likely associated with the altered bacterial species. Using a five-month symptom persistence GWI model in mice and whole-genome sequencing, we characterized the species-level dysbiosis and global metabolomics, along with heterogenous co-occurrence network analysis, to study the bacteriome-metabolomic association. Microbial analysis at the species level showed a significant alteration of beneficial bacterial species. The beta diversity of the global metabolomic profile showed distinct clustering due to the Western diet, along with the alteration of metabolites associated with lipid, amino acid, nucleotide, vitamin, and xenobiotic metabolism pathways. Network analysis showed novel associations of gut bacterial species with metabolites and biochemical pathways that could be used as biomarkers or therapeutic _targets to ameliorate symptom persistence in GW Veterans.
Keywords: chronic multisymptom illness; gut; metabolome; microbiome; whole-genome sequencing.
Conflict of interest statement
The authors declare no conflict of interest.
Figures
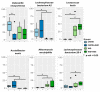
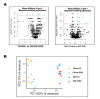
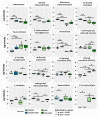
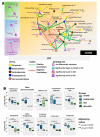
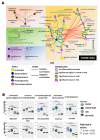
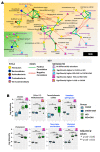
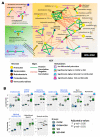
Similar articles
-
Obesity Worsens Gulf War Illness Symptom Persistence Pathology by Linking Altered Gut Microbiome Species to Long-Term Gastrointestinal, Hepatic, and Neuronal Inflammation in a Mouse Model.Nutrients. 2020 Sep 10;12(9):2764. doi: 10.3390/nu12092764. Nutrients. 2020. PMID: 32927823 Free PMC article.
-
Gulf War Illness Is Associated with Host Gut Microbiome Dysbiosis and Is Linked to Altered Species Abundance in Veterans from the BBRAIN Cohort.Int J Environ Res Public Health. 2024 Aug 21;21(8):1102. doi: 10.3390/ijerph21081102. Int J Environ Res Public Health. 2024. PMID: 39200711 Free PMC article.
-
Dysbiosis in gastrointestinal pathophysiology: Role of the gut microbiome in Gulf War Illness.J Cell Mol Med. 2023 Apr;27(7):891-905. doi: 10.1111/jcmm.17631. Epub 2023 Jan 30. J Cell Mol Med. 2023. PMID: 36716094 Free PMC article. Review.
-
Effects of a high fat diet on gut microbiome dysbiosis in a mouse model of Gulf War Illness.Sci Rep. 2020 Jun 12;10(1):9529. doi: 10.1038/s41598-020-66833-w. Sci Rep. 2020. PMID: 32533086 Free PMC article.
-
Host gut microbiome and potential therapeutics in Gulf War Illness: A short review.Life Sci. 2021 Sep 1;280:119717. doi: 10.1016/j.lfs.2021.119717. Epub 2021 Jun 15. Life Sci. 2021. PMID: 34139232 Review.
References
-
- Alhasson F., Das S., Seth R., Dattaroy D., Chandrashekaran V., Ryan C.N., Chan L.S., Testerman T., Burch J., Hofseth L.J., et al. Altered gut microbiome in a mouse model of Gulf War Illness causes neuroinflammation and intestinal injury via leaky gut and TLR4 activation. PLoS ONE. 2017;12:e0172914. doi: 10.1371/journal.pone.0172914. - DOI - PMC - PubMed
-
- Bose D., Mondal A., Saha P., Kimono D., Sarkar S., Seth R.K., Janulewicz P., Sullivan K., Horner R., Klimas N., et al. TLR Antagonism by Sparstolonin B Alters Microbial Signature and Modulates Gastrointestinal and Neuronal Inflammation in Gulf War Illness Preclinical Model. Brain Sci. 2020;10:532. doi: 10.3390/brainsci10080532. - DOI - PMC - PubMed
-
- Saha P., Skidmore P.T., Holland L.A., Mondal A., Bose D., Seth R.K., Sullivan K., Janulewicz P.A., Horner R., Klimas N., et al. Andrographolide Attenuates Gut-Brain-Axis Associated Pathology in Gulf War Illness by Modulating Bacteriome-Virome Associated Inflammation and Microglia-Neuron Proinflammatory Crosstalk. Brain Sci. 2021;11:905. doi: 10.3390/brainsci11070905. - DOI - PMC - PubMed
MeSH terms
Grants and funding
LinkOut - more resources
Full Text Sources