Comparison of decision tree with common machine learning models for prediction of biguanide and sulfonylurea poisoning in the United States: an analysis of the National Poison Data System
- PMID: 37024869
- PMCID: PMC10080923
- DOI: 10.1186/s12911-022-02095-y
Comparison of decision tree with common machine learning models for prediction of biguanide and sulfonylurea poisoning in the United States: an analysis of the National Poison Data System
Abstract
Background: Biguanides and sulfonylurea are two classes of anti-diabetic medications that have commonly been prescribed all around the world. Diagnosis of biguanide and sulfonylurea exposures is based on history taking and physical examination; thus, physicians might misdiagnose these two different clinical settings. We aimed to conduct a study to develop a model based on decision tree analysis to help physicians better diagnose these poisoning cases.
Methods: The National Poison Data System was used for this six-year retrospective cohort study.The decision tree model, common machine learning models multi layers perceptron, stochastic gradient descent (SGD), Adaboosting classiefier, linear support vector machine and ensembling methods including bagging, voting and stacking methods were used. The confusion matrix, precision, recall, specificity, f1-score, and accuracy were reported to evaluate the model's performance.
Results: Of 6183 participants, 3336 patients (54.0%) were identified as biguanides exposures, and the remaining were those with sulfonylureas exposures. The decision tree model showed that the most important clinical findings defining biguanide and sulfonylurea exposures were hypoglycemia, abdominal pain, acidosis, diaphoresis, tremor, vomiting, diarrhea, age, and reasons for exposure. The specificity, precision, recall, f1-score, and accuracy of all models were greater than 86%, 89%, 88%, and 88%, respectively. The lowest values belong to SGD model. The decision tree model has a sensitivity (recall) of 93.3%, specificity of 92.8%, precision of 93.4%, f1_score of 93.3%, and accuracy of 93.3%.
Conclusion: Our results indicated that machine learning methods including decision tree and ensembling methods provide a precise prediction model to diagnose biguanides and sulfonylureas exposure.
Keywords: Biguanide; Decision tree; NPDS; National Poison Data System; Overdose; Sulfonylurea.
© 2023. The Author(s).
Conflict of interest statement
The authors declare that they have no conflict of interest.
Figures
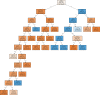
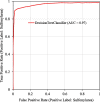
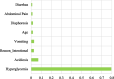
Similar articles
-
Distinguishing characteristics of exposure to biguanide and sulfonylurea anti-diabetic medications in the United States.Am J Emerg Med. 2022 Jun;56:171-177. doi: 10.1016/j.ajem.2022.03.023. Epub 2022 Mar 18. Am J Emerg Med. 2022. PMID: 35398707
-
Utility of artificial intelligence to identify antihyperglycemic agents poisoning in the USA: introducing a practical web application using National Poison Data System (NPDS).Environ Sci Pollut Res Int. 2023 Apr;30(20):57801-57810. doi: 10.1007/s11356-023-26605-1. Epub 2023 Mar 27. Environ Sci Pollut Res Int. 2023. PMID: 36973614
-
Utility of support vector machine and decision tree to identify the prognosis of metformin poisoning in the United States: analysis of National Poisoning Data System.BMC Pharmacol Toxicol. 2022 Jul 13;23(1):49. doi: 10.1186/s40360-022-00588-0. BMC Pharmacol Toxicol. 2022. PMID: 35831909 Free PMC article.
-
Oral hypoglycemic agents.Med Clin North Am. 1988 Nov;72(6):1323-35. doi: 10.1016/s0025-7125(16)30709-x. Med Clin North Am. 1988. PMID: 3054355 Review.
-
Home management of pediatric sulfonylurea ingestions.Clin Toxicol (Phila). 2022 Nov;60(11):1235-1239. doi: 10.1080/15563650.2022.2125875. Epub 2022 Oct 25. Clin Toxicol (Phila). 2022. PMID: 36282178 Review.
Cited by
-
Machine learning algorithms able to predict the prognosis of gastric cancer patients treated with immune checkpoint inhibitors.World J Gastroenterol. 2024 Oct 28;30(40):4354-4366. doi: 10.3748/wjg.v30.i40.4354. World J Gastroenterol. 2024. PMID: 39494097 Free PMC article.
-
Prediction of naloxone dose in opioids toxicity based on machine learning techniques (artificial intelligence).Daru. 2024 Dec;32(2):495-513. doi: 10.1007/s40199-024-00518-x. Epub 2024 May 21. Daru. 2024. PMID: 38771458
References
MeSH terms
Substances
LinkOut - more resources
Full Text Sources