The Relationship Between Lockdowns and Video Game Playtime: Multilevel Time-Series Analysis Using Massive-Scale Data Telemetry
- PMID: 37938889
- PMCID: PMC10666013
- DOI: 10.2196/40190
The Relationship Between Lockdowns and Video Game Playtime: Multilevel Time-Series Analysis Using Massive-Scale Data Telemetry
Abstract
Background: COVID-19 led governments worldwide to enact a variety of containment and closure policies. Substantial attention has been directed toward the idea that these public health measures may have unanticipated negative side effects. One proposed effect relates to video games. There is a nascent evidence base suggesting that individuals played video games for longer and in a more disordered manner during lockdowns and school closures specifically. These increases are commonly framed as a potential health concern in relation to disordered gaming. However, the evidence base regarding changes in gaming during the COVID-19 pandemic is based on self-report and, thus, is susceptible to bias. Therefore, it is unclear what the true consequences of lockdowns were for gaming behavior worldwide.
Objective: The primary objective of this study was to estimate whether any specific lockdown policy led to meaningful increases in the amount of time individuals spent playing video games.
Methods: Rather than relying on self-report, we used >251 billion hours of raw gameplay telemetry data from 184 separate countries to assess the behavioral correlates of COVID-19-related policy decisions. A multilevel model estimated the impact of varying enforcement levels of 8 containment and closure policies on the amount of time that individual users spent in-game. Similar models estimated the impact of policy on overall playtime and the number of users within a country.
Results: No lockdown policy can explain substantial variance in playtime per gamer. School closures were uniquely associated with meaningful increases in total playtime within a country (r2=0.048). However, this was associated with increases in the number of unique individuals playing games (r2=0.057) rather than increases in playtime per gamer (r2<0.001).
Conclusions: Previous work using self-report data has suggested that important increases in heavy gaming may occur during pandemics because of containment and closure ("lockdown") procedures. This study contrasts with the previous evidence base and finds no evidence of such a relationship. It suggests that significant further work is needed before increases in disordered or heavy gaming are considered when planning public health policies for pandemic preparedness.
Keywords: COVID-19; big data; disordered; disordered gaming; gaming; lockdown; lockdown policy; pandemic; playing; playtime; policy; public health; side effects; time; video games.
©David Zendle, Catherine Flick, Darel Halgarth, Nick Ballou, Joe Cutting, Anders Drachen. Originally published in the Journal of Medical Internet Research (https://www.jmir.org), 08.11.2023.
Conflict of interest statement
Conflicts of Interest: Data for this study were provided by Unity Technologies. Unity Technologies played no role in the design of the study, its reporting, or its execution. No funds were disbursed to the research team for the work incorporated into this manuscript. This includes data access fees for the serverless data warehouse from which the data under analysis were accessed, which were paid by the lead author out of a research stipend he receives from his host institution. Access to the data used in this study was contingent on a data-sharing agreement between Unity Technologies and DZ’s host institution. Legal approval was sought from Unity for the sharing of the data in this paper before its submission for peer review. DZ has never received any form of funding from the games industry. He has worked as a paid consultant for governments seeking to understand the effects of video games and gambling. He has worked as an expert witness in cases relating to the video game industry but has never represented the games industry legally or been formally affiliated with any games industry body in any way. DZ has been involved in brokering data-sharing agreements with industry stakeholders in the past. He acknowledges that such data-sharing agreements constitute a conflict of interest as important as financial awards and wishes to highlight that he has used such data brokerage in ways that are likely to give him indirect financial advantage: he has used them as evidence for excellence in promotion applications; he has used them as evidence in grant applications. No such applications have been funded at the time of writing this manuscript. DZ is a member of the Advisory Board for Safer Gambling, a statutory body whose remit is to provide independent advice to the UK Gambling Commission. DZ is the recipient of an Academic Forum for the Study of Gambling Major Exploratory Grant that is derived from “regulatory settlements applied for socially responsible purposes” received by the UK Gambling Commission and administered by Gambling Research Exchange Ontario (GREO). He has no further conflicts to declare. CF has received funding from the Tides Foundation on the recommendation of the Unity Charitable Fund (grant TF2201-105180) for a separate project. AD has previously worked in a paid capacity within the video games industry as a game analytics consultant. He has worked on multiple industry-focused projects with a focus on knowledge transfer. He has received funding from the Tides Foundation on the recommendation of the Unity Charitable Fund (grant TF2201-105180) for a separate project.
Figures
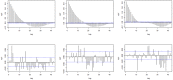
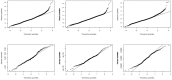
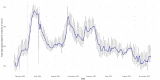
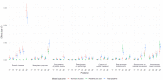
Similar articles
-
Addictive Internet Gaming Usage among Korean Adolescents before and after the Outbreak of the COVID-19 Pandemic: A Comparison of the Latent Profiles in 2018 and 2020.Int J Environ Res Public Health. 2021 Jul 7;18(14):7275. doi: 10.3390/ijerph18147275. Int J Environ Res Public Health. 2021. PMID: 34299725 Free PMC article.
-
Gaming in Pandemic Times: An International Survey Assessing the Effects of COVID-19 Lockdowns on Young Video Gamers' Health.Int J Environ Res Public Health. 2023 Sep 28;20(19):6855. doi: 10.3390/ijerph20196855. Int J Environ Res Public Health. 2023. PMID: 37835124 Free PMC article.
-
Gaming among Children and Adolescents during the COVID-19 Lockdown: The Role of Parents in Time Spent on Video Games and Gaming Disorder Symptoms.Int J Environ Res Public Health. 2021 Jun 21;18(12):6642. doi: 10.3390/ijerph18126642. Int J Environ Res Public Health. 2021. PMID: 34205533 Free PMC article.
-
The Effects of Playing Video Games on Stress, Anxiety, Depression, Loneliness, and Gaming Disorder During the Early Stages of the COVID-19 Pandemic: PRISMA Systematic Review.Cyberpsychol Behav Soc Netw. 2022 Jun;25(6):334-354. doi: 10.1089/cyber.2021.0252. Epub 2022 May 27. Cyberpsychol Behav Soc Netw. 2022. PMID: 35639118 Review.
-
Video Game Use in the Treatment of Amblyopia: Weighing the Risks of Addiction.Yale J Biol Med. 2015 Sep 3;88(3):309-17. eCollection 2015 Sep. Yale J Biol Med. 2015. PMID: 26339215 Free PMC article. Review.
Cited by
-
Improving Social and Personal Rhythm Dysregulation in Young and Old Adults with Bipolar Disorder: Post-Hoc Analysis of a Feasibility Randomized Controlled Trial Using Virtual Reality-Based Intervention.J Clin Med. 2024 Jun 27;13(13):3786. doi: 10.3390/jcm13133786. J Clin Med. 2024. PMID: 38999351 Free PMC article.
-
When Games Influence Words: Gaming Addiction among College Students Increases Verbal Aggression through Risk-Biased Drifting in Decision-Making.Behav Sci (Basel). 2024 Aug 11;14(8):699. doi: 10.3390/bs14080699. Behav Sci (Basel). 2024. PMID: 39199095 Free PMC article.
-
The Stability of Social and Behavioral Rhythms and Unexpected Low Rate of Relevant Depressive Symptoms in Old Adults during the COVID-19 Pandemic.J Clin Med. 2024 Mar 29;13(7):2005. doi: 10.3390/jcm13072005. J Clin Med. 2024. PMID: 38610769 Free PMC article.
References
-
- Rader B, White LF, Burns MR, Chen J, Brilliant J, Cohen J, Shaman J, Brilliant L, Kraemer MU, Hawkins JB, Scarpino SV, Astley CM, Brownstein JS. Mask-wearing and control of SARS-CoV-2 transmission in the USA: a cross-sectional study. Lancet Digit Health. 2021 Mar;3(3):e148–57. doi: 10.1016/S2589-7500(20)30293-4. https://linkinghub.elsevier.com/retrieve/pii/S2589-7500(20)30293-4 S2589-7500(20)30293-4 - DOI - PMC - PubMed
-
- Duan Y, Shi J, Wang Z, Zhou S, Jin Y, Zheng Z-J. Disparities in COVID-19 vaccination among low-, middle-, and high-income countries: the mediating role of vaccination policy. Vaccines (Basel) 2021 Aug 14;9(8):905. doi: 10.3390/vaccines9080905. https://www.mdpi.com/resolver?pii=vaccines9080905 vaccines9080905 - DOI - PMC - PubMed
-
- Piguillem F, Shi L. Optimal Covid-19 quarantine and testing policies. Econ J. 2022 Oct;132(647):2534–62. doi: 10.1093/ej/ueac026. - DOI
-
- Faria-E-Castro M. Fiscal policy during a pandemic. J Econ Dyn Control. 2021 Apr;125:104088. doi: 10.1016/j.jedc.2021.104088. https://europepmc.org/abstract/MED/36540418 S0165-1889(21)00023-3 - DOI - PMC - PubMed
-
- Hale T, Angrist N, Goldszmidt R, Kira B, Petherick A, Phillips T, Webster S, Cameron-Blake E, Hallas L, Majumdar S, Tatlow H. A global panel database of pandemic policies (Oxford COVID-19 Government Response Tracker) Nat Hum Behav. 2021 Apr 08;5(4):529–38. doi: 10.1038/s41562-021-01079-8.10.1038/s41562-021-01079-8 - DOI - PubMed
Publication types
MeSH terms
LinkOut - more resources
Full Text Sources
Medical