Large language models assisted multi-effect variants mining on cerebral cavernous malformation familial whole genome sequencing
- PMID: 38352937
- PMCID: PMC10861960
- DOI: 10.1016/j.csbj.2024.01.014
Large language models assisted multi-effect variants mining on cerebral cavernous malformation familial whole genome sequencing
Abstract
Cerebral cavernous malformation (CCM) is a polygenic disease with intricate genetic interactions contributing to quantitative pathogenesis across multiple factors. The principal pathogenic genes of CCM, specifically KRIT1, CCM2, and PDCD10, have been reported, accompanied by a growing wealth of genetic data related to mutations. Furthermore, numerous other molecules associated with CCM have been unearthed. However, tackling such massive volumes of unstructured data remains challenging until the advent of advanced large language models. In this study, we developed an automated analytical pipeline specialized in single nucleotide variants (SNVs) related biomedical text analysis called BRLM. To facilitate this, BioBERT was employed to vectorize the rich information of SNVs, while a deep residue network was used to discriminate the classes of the SNVs. BRLM was initially constructed on mutations from 12 different types of TCGA cancers, achieving an accuracy exceeding 99%. It was further examined for CCM mutations in familial sequencing data analysis, highlighting an upstream master regulator gene fibroblast growth factor 1 (FGF1). With multi-omics characterization and validation in biological function, FGF1 demonstrated to play a significant role in the development of CCMs, which proved the effectiveness of our model. The BRLM web server is available at http://1.117.230.196.
Keywords: Cerebral cavernous malformation; Deep learning; Large language model; Natural language processing; Whole genome sequencing.
© 2024 The Authors.
Conflict of interest statement
The authors declare that they have no known competing financial interests or personal relationships that could have appeared to influence the work reported in this paper.
Figures
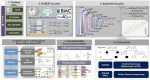
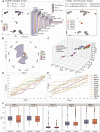
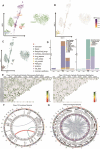
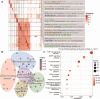
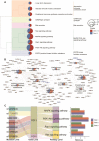
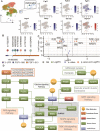
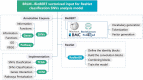
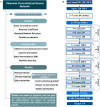
Similar articles
-
A single-center study on 140 patients with cerebral cavernous malformations: 28 new pathogenic variants and functional characterization of a PDCD10 large deletion.Hum Mutat. 2018 Dec;39(12):1885-1900. doi: 10.1002/humu.23629. Epub 2018 Sep 24. Hum Mutat. 2018. PMID: 30161288
-
High-throughput sequencing of the entire genomic regions of CCM1/KRIT1, CCM2 and CCM3/PDCD10 to search for pathogenic deep-intronic splice mutations in cerebral cavernous malformations.Eur J Med Genet. 2017 Sep;60(9):479-484. doi: 10.1016/j.ejmg.2017.06.007. Epub 2017 Jun 20. Eur J Med Genet. 2017. PMID: 28645800
-
[Gene mutations in patients with hereditary cavernous malformations].Zh Nevrol Psikhiatr Im S S Korsakova. 2017;117(6):66-72. doi: 10.17116/jnevro20171176166-72. Zh Nevrol Psikhiatr Im S S Korsakova. 2017. PMID: 28745674 Russian.
-
Cerebral Cavernous Malformations: Review of the Genetic and Protein-Protein Interactions Resulting in Disease Pathogenesis.Front Surg. 2016 Nov 14;3:60. doi: 10.3389/fsurg.2016.00060. eCollection 2016. Front Surg. 2016. PMID: 27896269 Free PMC article. Review.
-
Cerebral cavernous malformation: new molecular and clinical insights.J Med Genet. 2006 Sep;43(9):716-21. doi: 10.1136/jmg.2006.041079. Epub 2006 Mar 29. J Med Genet. 2006. PMID: 16571644 Free PMC article. Review.
Cited by
-
Large language models in neurosurgery: a systematic review and meta-analysis.Acta Neurochir (Wien). 2024 Nov 23;166(1):475. doi: 10.1007/s00701-024-06372-9. Acta Neurochir (Wien). 2024. PMID: 39579215 Review.
References
-
- Afzal S., Asim M., Javed A.R., Beg M.O., Baker T. Urldeepdetect: a deep learning approach for detecting malicious urls using semantic vector models. J Netw Syst Manag. 2021;29:1–27.
-
- Atkinson E., Dickman R. Growth factors and their peptide mimetics for treatment of traumatic brain injury. Bioorg Med Chem. 2023 - PubMed
LinkOut - more resources
Full Text Sources