Potential of Unmanned Aerial Vehicle Red-Green-Blue Images for Detecting Needle Pests: A Case Study with Erannis jacobsoni Djak (Lepidoptera, Geometridae)
- PMID: 38535368
- PMCID: PMC10971561
- DOI: 10.3390/insects15030172
Potential of Unmanned Aerial Vehicle Red-Green-Blue Images for Detecting Needle Pests: A Case Study with Erannis jacobsoni Djak (Lepidoptera, Geometridae)
Abstract
Erannis jacobsoni Djak (Lepidoptera, Geometridae) is a leaf-feeding pest unique to Mongolia. Outbreaks of this pest can cause larch needles to shed slowly from the top until they die, leading to a serious imbalance in the forest ecosystem. In this work, to address the need for the low-cost, fast, and effective identification of this pest, we used field survey indicators and UAV images of larch forests in Binder, Khentii, Mongolia, a typical site of Erannis jacobsoni Djak pest outbreaks, as the base data, calculated relevant multispectral and red-green-blue (RGB) features, used a successive projections algorithm (SPA) to extract features that are sensitive to the level of pest damage, and constructed a recognition model of Erannis jacobsoni Djak pest damage by combining patterns in the RGB vegetation indices and texture features (RGBVI&TF) with the help of random forest (RF) and convolutional neural network (CNN) algorithms. The results were compared and evaluated with multispectral vegetation indices (MSVI) to explore the potential of UAV RGB images in identifying needle pests. The results show that the sensitive features extracted based on SPA can adequately capture the changes in the forest appearance parameters such as the leaf loss rate and the colour of the larch canopy under pest damage conditions and can be used as effective input variables for the model. The RGBVI&TF-RF440 and RGBVI&TF-CNN740 models have the best performance, with their overall accuracy reaching more than 85%, which is a significant improvement compared with that of the RGBVI model, and their accuracy is similar to that of the MSVI model. This low-cost and high-efficiency method can excel in the identification of Erannis jacobsoni Djak-infested regions in small areas and can provide an important experimental theoretical basis for subsequent large-scale forest pest monitoring with a high spatiotemporal resolution.
Keywords: Erannis jacobsoni Djak; RGB texture features; RGB vegetation indices; UAV images; damage level recognition; machine learning.
Conflict of interest statement
The authors declare no conflict of interest.
Figures
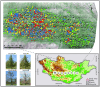
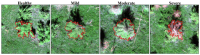
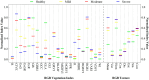
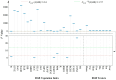
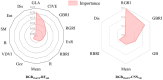
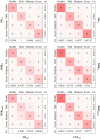
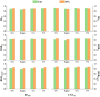
Similar articles
-
Detecting Pest-Infested Forest Damage through Multispectral Satellite Imagery and Improved UNet+.Sensors (Basel). 2022 Sep 30;22(19):7440. doi: 10.3390/s22197440. Sensors (Basel). 2022. PMID: 36236538 Free PMC article.
-
Cotton Yield Estimation Based on Vegetation Indices and Texture Features Derived From RGB Image.Front Plant Sci. 2022 Jun 15;13:925986. doi: 10.3389/fpls.2022.925986. eCollection 2022. Front Plant Sci. 2022. PMID: 35783985 Free PMC article.
-
Application of UAV Multisensor Data and Ensemble Approach for High-Throughput Estimation of Maize Phenotyping Traits.Plant Phenomics. 2022 Aug 27;2022:9802585. doi: 10.34133/2022/9802585. eCollection 2022. Plant Phenomics. 2022. PMID: 36158531 Free PMC article.
-
Combining spectral and texture feature of UAV image with plant height to improve LAI estimation of winter wheat at jointing stage.Front Plant Sci. 2024 Jan 3;14:1272049. doi: 10.3389/fpls.2023.1272049. eCollection 2023. Front Plant Sci. 2024. PMID: 38235191 Free PMC article.
-
Estimation of Rice Aboveground Biomass by Combining Canopy Spectral Reflectance and Unmanned Aerial Vehicle-Based Red Green Blue Imagery Data.Front Plant Sci. 2022 May 27;13:903643. doi: 10.3389/fpls.2022.903643. eCollection 2022. Front Plant Sci. 2022. PMID: 35712565 Free PMC article.
References
-
- Bai L., Huang X., Dashzebeg G., Ariunaa M., Nanzad T., Dorjsuren A., Bao G., Tong S., Bao Y., Yin S., et al. Estimation of the population density of Erannis jacobsoni (Lepidoptera: Geometridae) based on hyperspectral features. Acta Entomol. Sin. 2021;64:711–721.
-
- Huang X., Xie Y., Bao Y., Bao G., Qing S., Bao Y. Estimation of leaf loss rate in larch infested with Erannis jacobsoni Djak based on differential spectral continuous wavelet coefficients. Spectrosc. Spectr. Anal. 2019;39:2732–2738.
-
- Huang X., Xie Y., Bao Y., Dorjsuren A. Suitable distribution areas of Jas’s larch inchworm in Mongolia Plateau. J. Northwest A F Univ. Nat. Sci. Ed. 2018;46:98–106.
-
- Huang X., Xie Y., Bao Y. Spectral detection of damaged level of larch affected by Jas’s larch inchworm. Spectrosc. Spectr. Anal. 2018;38:905–911.
-
- Tian M., Ban S., Yuan T., Wang Y., Ma C., Li L. Monitoring of rice damage by rice leaf roller using UAV-based remote sensing. Acta Agric. Shanghai. 2020;36:132–137.
Grants and funding
LinkOut - more resources
Full Text Sources
Miscellaneous