Metapopulation model of phage therapy of an acute Pseudomonas aeruginosa lung infection
- PMID: 39230264
- PMCID: PMC11562898
- DOI: 10.1128/msystems.00171-24
Metapopulation model of phage therapy of an acute Pseudomonas aeruginosa lung infection
Abstract
Infections caused by multidrug resistant (MDR) pathogenic bacteria are a global health threat. Bacteriophages ("phage") are increasingly used as alternative or last-resort therapeutics to treat patients infected by MDR bacteria. However, the therapeutic outcomes of phage therapy may be limited by the emergence of phage resistance during treatment and/or by physical constraints that impede phage-bacteria interactions in vivo. In this work, we evaluate the role of lung spatial structure on the efficacy of phage therapy for Pseudomonas aeruginosa infections. To do so, we developed a spatially structured metapopulation network model based on the geometry of the bronchial tree, including host innate immune responses and the emergence of phage-resistant bacterial mutants. We model the ecological interactions between bacteria, phage, and the host innate immune system at the airway (node) level. The model predicts the synergistic elimination of a P. aeruginosa infection due to the combined effects of phage and neutrophils, given the sufficient innate immune activity and efficient phage-induced lysis. The metapopulation model simulations also predict that MDR bacteria are cleared faster at distal nodes of the bronchial tree. Notably, image analysis of lung tissue time series from wild-type and lymphocyte-depleted mice revealed a concordant, statistically significant pattern: infection intensity cleared in the bottom before the top of the lungs. Overall, the combined use of simulations and image analysis of in vivo experiments further supports the use of phage therapy for treating acute lung infections caused by P. aeruginosa, while highlighting potential limits to therapy in a spatially structured environment given impaired innate immune responses and/or inefficient phage-induced lysis.
Importance: Phage therapy is increasingly employed as a compassionate treatment for severe infections caused by multidrug-resistant (MDR) bacteria. However, the mixed outcomes observed in larger clinical studies highlight a gap in understanding when phage therapy succeeds or fails. Previous research from our team, using in vivo experiments and single-compartment mathematical models, demonstrated the synergistic clearance of acute P. aeruginosa pneumonia by phage and neutrophils despite the emergence of phage-resistant bacteria. In fact, the lung environment is highly structured, prompting the question of whether immunophage synergy explains the curative treatment of P. aeruginosa when incorporating realistic physical connectivity. To address this, we developed a metapopulation network model mimicking the lung branching structure to assess phage therapy efficacy for MDR P. aeruginosa pneumonia. The model predicts the synergistic elimination of P. aeruginosa by phage and neutrophils but emphasizes potential challenges in spatially structured environments, suggesting that higher innate immune levels may be required for successful bacterial clearance. Model simulations reveal a spatial pattern in pathogen clearance where P. aeruginosa are cleared faster at distal nodes of the bronchial tree than in primary nodes. Interestingly, image analysis of infected mice reveals a concordant and statistically significant pattern: infection intensity clears in the bottom before the top of the lungs. The combined use of modeling and image analysis supports the application of phage therapy for acute P. aeruginosa pneumonia while emphasizing potential challenges to curative success in spatially structured in vivo environments, including impaired innate immune responses and reduced phage efficacy.
Keywords: Pseudomonas aeruginosa; antibiotic resistance; antimicrobial agents; bacteriophage therapy; infectious disease; innate immunity; lung infection; mathematical modeling; microbial ecology; virology.
Conflict of interest statement
The authors declare no conflict of interest.
Figures
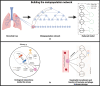
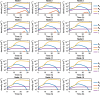
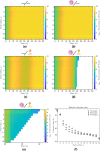
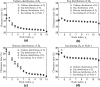
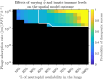
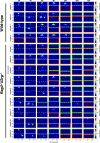
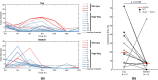
Update of
-
Metapopulation model of phage therapy of an acute Pseudomonas aeruginosa lung infection.bioRxiv [Preprint]. 2024 Jan 31:2024.01.31.578251. doi: 10.1101/2024.01.31.578251. bioRxiv. 2024. Update in: mSystems. 2024 Oct 22;9(10):e0017124. doi: 10.1128/msystems.00171-24 PMID: 38352502 Free PMC article. Updated. Preprint.
Similar articles
-
Metapopulation model of phage therapy of an acute Pseudomonas aeruginosa lung infection.bioRxiv [Preprint]. 2024 Jan 31:2024.01.31.578251. doi: 10.1101/2024.01.31.578251. bioRxiv. 2024. Update in: mSystems. 2024 Oct 22;9(10):e0017124. doi: 10.1128/msystems.00171-24 PMID: 38352502 Free PMC article. Updated. Preprint.
-
Synergy between the Host Immune System and Bacteriophage Is Essential for Successful Phage Therapy against an Acute Respiratory Pathogen.Cell Host Microbe. 2017 Jul 12;22(1):38-47.e4. doi: 10.1016/j.chom.2017.06.018. Cell Host Microbe. 2017. PMID: 28704651
-
Bacteriophage Therapy Increases Complement-Mediated Lysis of Bacteria and Enhances Bacterial Clearance After Acute Lung Infection With Multidrug-Resistant Pseudomonas aeruginosa.J Infect Dis. 2019 Apr 16;219(9):1439-1447. doi: 10.1093/infdis/jiy678. J Infect Dis. 2019. PMID: 30476337
-
The potential of phage therapy in cystic fibrosis: Essential human-bacterial-phage interactions and delivery considerations for use in Pseudomonas aeruginosa-infected airways.J Cyst Fibros. 2017 Nov;16(6):663-670. doi: 10.1016/j.jcf.2017.06.012. Epub 2017 Jul 15. J Cyst Fibros. 2017. PMID: 28720345 Review.
-
Pseudomonas aeruginosa Bacteriophages and Their Clinical Applications.Viruses. 2024 Jun 29;16(7):1051. doi: 10.3390/v16071051. Viruses. 2024. PMID: 39066214 Free PMC article. Review.
Cited by
-
Multi-strain phage induced clearance of bacterial infections.bioRxiv [Preprint]. 2024 Sep 7:2024.09.07.611814. doi: 10.1101/2024.09.07.611814. bioRxiv. 2024. PMID: 39282405 Free PMC article. Preprint.
References
-
- World Health Organization . 2022. 2021 antibacterial agents in clinical and preclinical development: an overview and analysis. World Health Organization.
MeSH terms
Grants and funding
LinkOut - more resources
Full Text Sources
Miscellaneous