The Effects of Soil Depth on the Structure of Microbial Communities in Agricultural Soils in Iowa, USA
- PMID: 33310710
- PMCID: PMC7851703
- DOI: 10.1128/AEM.02673-20
The Effects of Soil Depth on the Structure of Microbial Communities in Agricultural Soils in Iowa, USA
Abstract
This study investigated the differences in microbial community abundance, composition and diversity throughout the depth profiles in soils collected from corn and soybean fields in lowa, USA using 16S rRNA amplicon sequencing. The results revealed decreased richness and diversity in microbial communities at increasing soil depth. Soil microbial community composition differed due to crop type only in the top 60 cm and due to location only in the top 90 cm. While the relative abundance of most phyla decreased in deep soils, the relative abundance of the phylum Proteobacteria increased and dominated agricultural soils below the depth of 90 cm. Although soil depth was the most important factor shaping microbial communities, edaphic factors including soil organic matter, soil bulk density and the length of time that deep soils were saturated with water were all significant factors explaining the variation in soil microbial community composition. Soil organic matter showed the highest correlation with the exponential decrease in bacterial abundance with depth. A greater understanding of how soil depth influences the diversity and composition of soil microbial communities is vital for guiding sampling approaches in agricultural soils where plant roots extend beyond the upper soil profile. In the long term a greater knowledge of the influence of depth on microbial communities should contribute to new strategies that enhance the sustainability of soil which is a precious resource for food security.IMPORTANCE Determining how microbial properties change across different soils and within the soil depth profile, will be potentially beneficial to understanding the long-term processes that are involved in the health of agricultural ecosystems. Most literature on soil microbes has been restricted to the easily accessible surface soils. However, deep soils are important in soil formation, carbon sequestration, and in providing nutrients and water for plants. In the most productive agricultural systems in the USA where soybean and corn are grown, crop plant roots extend into the deeper regions of soils (> 100 cm), but little is known about the taxonomic diversity or the factors that shape deep soil microbial communities. The findings reported here highlight the importance of soil depth in shaping microbial communities, provide new information about edaphic factors that influence the deep soil communities and reveal more detailed information on taxa that exist in deep agricultural soils.
Copyright © 2020 Hao et al.
Figures
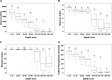
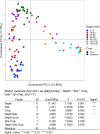
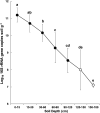
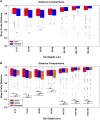
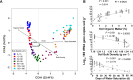
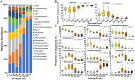
Similar articles
-
Microenvironment heterogeneity affected by anthropogenic wildfire-perturbed soil mediates bacterial community in Pinus tabulaeformis forests.Front Microbiol. 2024 Jul 9;15:1415726. doi: 10.3389/fmicb.2024.1415726. eCollection 2024. Front Microbiol. 2024. PMID: 39044951 Free PMC article.
-
Ecological and Genomic Attributes of Novel Bacterial Taxa That Thrive in Subsurface Soil Horizons.mBio. 2019 Oct 1;10(5):e01318-19. doi: 10.1128/mBio.01318-19. mBio. 2019. PMID: 31575762 Free PMC article.
-
Microbial Community and Functional Structure Significantly Varied among Distinct Types of Paddy Soils But Responded Differently along Gradients of Soil Depth Layers.Front Microbiol. 2017 May 29;8:945. doi: 10.3389/fmicb.2017.00945. eCollection 2017. Front Microbiol. 2017. PMID: 28611747 Free PMC article.
-
Trends in Microbial Community Composition and Function by Soil Depth.Microorganisms. 2022 Feb 28;10(3):540. doi: 10.3390/microorganisms10030540. Microorganisms. 2022. PMID: 35336115 Free PMC article. Review.
-
Links Among Crop Diversification, Microbial Diversity, and Soil Organic Carbon: Mini Review and Case Studies.Front Microbiol. 2022 Apr 25;13:854247. doi: 10.3389/fmicb.2022.854247. eCollection 2022. Front Microbiol. 2022. PMID: 35547111 Free PMC article. Review.
Cited by
-
Bacterial but Not Fungal Rhizosphere Community Composition Differ among Perennial Grass Ecotypes under Abiotic Environmental Stress.Microbiol Spectr. 2022 Jun 29;10(3):e0239121. doi: 10.1128/spectrum.02391-21. Epub 2022 Apr 20. Microbiol Spectr. 2022. PMID: 35442065 Free PMC article.
-
Ranking environmental and edaphic attributes driving soil microbial community structure and activity with special attention to spatial and temporal scales.mLife. 2024 Mar 26;3(1):21-41. doi: 10.1002/mlf2.12116. eCollection 2024 Mar. mLife. 2024. PMID: 38827504 Free PMC article. Review.
-
Vertical differences in carbon metabolic diversity and dominant flora of soil bacterial communities in farmlands.Sci Rep. 2024 Apr 24;14(1):9445. doi: 10.1038/s41598-024-60142-2. Sci Rep. 2024. PMID: 38658691 Free PMC article.
-
Soil pH: a key edaphic factor regulating distribution and functions of bacterial community along vertical soil profiles in red soil of pomelo orchard.BMC Microbiol. 2022 Feb 2;22(1):38. doi: 10.1186/s12866-022-02452-x. BMC Microbiol. 2022. PMID: 35109809 Free PMC article.
-
Metagenomic Sequencing of Multiple Soil Horizons and Sites in Close Vicinity Revealed Novel Secondary Metabolite Diversity.mSystems. 2021 Oct 26;6(5):e0101821. doi: 10.1128/mSystems.01018-21. Epub 2021 Oct 12. mSystems. 2021. PMID: 34636675 Free PMC article.
References
-
- Cotrufo MF, Wallenstein MD, Boot CM, Denef K, Paul E. 2013. The Microbial Efficiency-Matrix Stabilization (MEMS) framework integrates plant litter decomposition with soil organic matter stabilization: do labile plant inputs form stable soil organic matter? Glob Chang Biol 19:988–995. doi:10.1111/gcb.12113. - DOI - PubMed
LinkOut - more resources
Full Text Sources
Other Literature Sources