Metabolomics Simultaneously Derives Benchmark Dose Estimates and Discovers Metabolic Biotransformations in a Rat Bioassay
- PMID: 38842447
- PMCID: PMC11187623
- DOI: 10.1021/acs.chemrestox.4c00002
Metabolomics Simultaneously Derives Benchmark Dose Estimates and Discovers Metabolic Biotransformations in a Rat Bioassay
Abstract
Benchmark dose (BMD) modeling estimates the dose of a chemical that causes a perturbation from baseline. Transcriptional BMDs have been shown to be relatively consistent with apical end point BMDs, opening the door to using molecular BMDs to derive human health-based guidance values for chemical exposure. Metabolomics measures the responses of small-molecule endogenous metabolites to chemical exposure, complementing transcriptomics by characterizing downstream molecular phenotypes that are more closely associated with apical end points. The aim of this study was to apply BMD modeling to in vivo metabolomics data, to compare metabolic BMDs to both transcriptional and apical end point BMDs. This builds upon our previous application of transcriptomics and BMD modeling to a 5-day rat study of triphenyl phosphate (TPhP), applying metabolomics to the same archived tissues. Specifically, liver from rats exposed to five doses of TPhP was investigated using liquid chromatography-mass spectrometry and 1H nuclear magnetic resonance spectroscopy-based metabolomics. Following the application of BMDExpress2 software, 2903 endogenous metabolic features yielded viable dose-response models, confirming a perturbation to the liver metabolome. Metabolic BMD estimates were similarly sensitive to transcriptional BMDs, and more sensitive than both clinical chemistry and apical end point BMDs. Pathway analysis of the multiomics data sets revealed a major effect of TPhP exposure on cholesterol (and downstream) pathways, consistent with clinical chemistry measurements. Additionally, the transcriptomics data indicated that TPhP activated xenobiotic metabolism pathways, which was confirmed by using the underexploited capability of metabolomics to detect xenobiotic-related compounds. Eleven biotransformation products of TPhP were discovered, and their levels were highly correlated with multiple xenobiotic metabolism genes. This work provides a case study showing how metabolomics and transcriptomics can estimate mechanistically anchored points-of-departure. Furthermore, the study demonstrates how metabolomics can also discover biotransformation products, which could be of value within a regulatory setting, for example, as an enhancement of OECD Test Guideline 417 (toxicokinetics).
Conflict of interest statement
The authors declare the following competing financial interest(s): Professors Mark Viant and John Colbourne are employees of the University of Birmingham. They are also Founders and Directors of Michabo Health Science Ltd., a spin-out company of the University of Birmingham that provides scientific consultancy services in New Approach Methodologies (NAMs) specialising in omics technologies and computational toxicology.
Figures
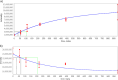
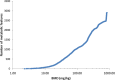
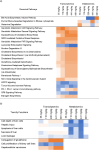
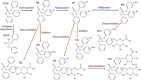
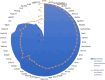
Similar articles
-
NIEHS Report on the In Vivo Repeat Dose Biological Potency Study of Perfluorohexanesulfonamide (CASRN 41997-13-1) in Sprague Dawley (Hsd:Sprague Dawley® SD®) Rats (Gavage Studies): NIEHS Report 10 [Internet].Research Triangle Park (NC): National Institute of Environmental Health Sciences; 2023 Mar. Research Triangle Park (NC): National Institute of Environmental Health Sciences; 2023 Mar. PMID: 37018438 Free Books & Documents. Review.
-
NIEHS Report on the In Vivo Repeat Dose Biological Potency Study of 6:1 Fluorotelomer Alcohol (CASRN 375-82-6) in Sprague Dawley (Hsd:Sprague Dawley® SD®) Rats (Gavage Studies): NIEHS Report 07 [Internet].Research Triangle Park (NC): National Institute of Environmental Health Sciences; 2023 Mar. Research Triangle Park (NC): National Institute of Environmental Health Sciences; 2023 Mar. PMID: 37018435 Free Books & Documents. Review.
-
NIEHS Report on the In Vivo Repeat Dose Biological Potency Study of 2,3-Benzofluorene (CASRN 243-17-4) in Sprague Dawley (Hsd:Sprague Dawley® SD®) Rats (Gavage Studies): NIEHS Report 09 [Internet].Research Triangle Park (NC): National Institute of Environmental Health Sciences; 2023 Mar. Research Triangle Park (NC): National Institute of Environmental Health Sciences; 2023 Mar. PMID: 37018437 Free Books & Documents. Review.
-
NIEHS Report on the In Vivo Repeat Dose Biological Potency Study of 1,1,2,2-Tetrahydroperfluoro-1-dodecanol (CASRN 865-86-1) in Sprague Dawley (Hsd:Sprague Dawley® SD®) Rats (Gavage Studies): NIEHS Report 08 [Internet].Research Triangle Park (NC): National Institute of Environmental Health Sciences; 2023 Mar. Research Triangle Park (NC): National Institute of Environmental Health Sciences; 2023 Mar. PMID: 37018436 Free Books & Documents. Review.
-
NTP Research Report on In Vivo Repeat Dose Biological Potency Study of Triphenyl Phosphate (CAS No. 115-86-6) in Male Sprague Dawley Rats (Hsd: Sprague Dawley SD) (Gavage Studies): Research Report 8 [Internet].Durham (NC): National Toxicology Program; 2018 Dec. Durham (NC): National Toxicology Program; 2018 Dec. PMID: 30589522 Free Books & Documents. Review.
References
-
- Farmahin R.; Williams A.; Kuo B.; Chepelev N. L.; Thomas R. S.; Barton-Maclaren T. S.; Curran I. H.; Nong A.; Wade M. G.; Yauk C. L. Recommended approaches in the application of toxicogenomics to derive points of departure for chemical risk assessment. Arch. Toxicol. 2017, 91 (5), 2045–2065. 10.1007/s00204-016-1886-5. - DOI - PMC - PubMed
-
- U.S.EPA . Benchmark dose technical guidance, EPA/100/R-12/001 June 2012. In Risk Assessment Forum; U.S. Environmental Protection Agency (EPA): Washington (DC), 2012.
MeSH terms
Substances
LinkOut - more resources
Full Text Sources